Figures & data
Figure 1. (a) The spatial distribution of the POI data and the road data. (b) Overview of the study area. (c) The spatial distribution of the POI kernel density. (d) The spatial distribution of three levels of the road network.
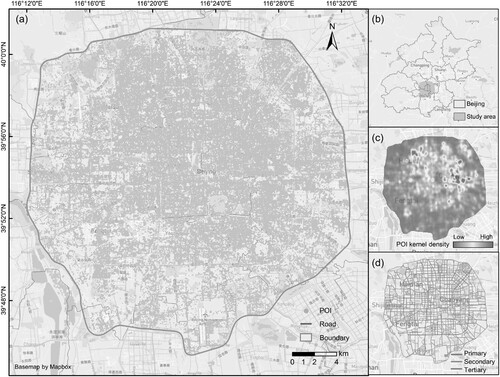
Figure 2. Rank-frequency plot for POI Sub-types. (a) Rank-frequency plot and (b) Rank-frequency log plot.
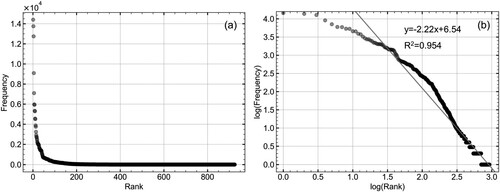
Figure 7. Schematic diagram of the geo-corpus construction approaches. (a) Approach A. (b) Approach B. (c) Approach C.
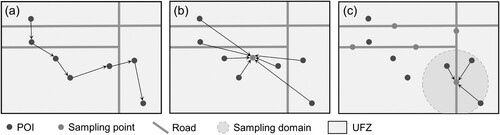
Table 1. Experiments design based on four combination modes.
Figure 11. The pairwise similarity between UFZs ranked by K-means-based cluster, and locations and remote sensing images of the six sample zones in cluster #4.
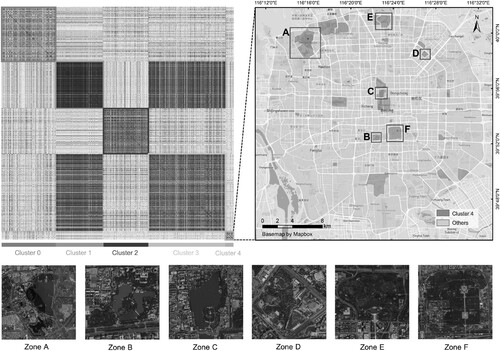
Figure 14. Classification results of UFZs. (0) Ground truth. (1) ∼ (15) Results of experiment 1 ∼ 15, and experiment 15 corresponds to our proposed method. Sparse zones refer to spatial units that are excluded from the study.
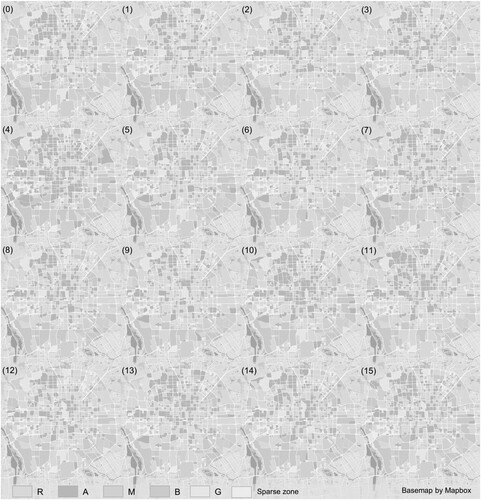
Table 2. Confusion matrix of classification results. (R: residential zone. A: administrative and public service zone. M: industrial zone. B: business zone. G: green space).
Table 3. Accuracy evaluation. (*: feature combination mode. R: residential zone. A: administrative and public service zone. M: industrial zone. B: business zone. G: green space).