Figures & data
Figure 1. The study area in Beijing and the segregation of traffic analysis zones. Abbreviations of places: CDG – Chedaogou, CQ – Caoqiao, GM – Guomao, JRS – Jinrong Street, OFP – Olympic Forest Park, PKU – Peking University, SP – the Summer Palace, TH – Temple of Heaven, THU – Tsinghua University, ZGC – Zhongguancun.
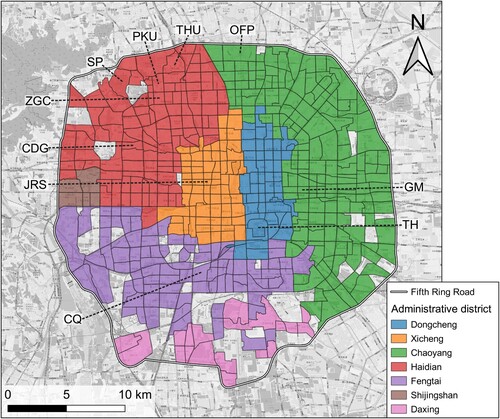
Figure 3. The dimensionality reduction of three feature embeddings, (a) activity dynamic features, (b) mobility interaction features, (c) activity semantic features. See for the abbreviations of the places.
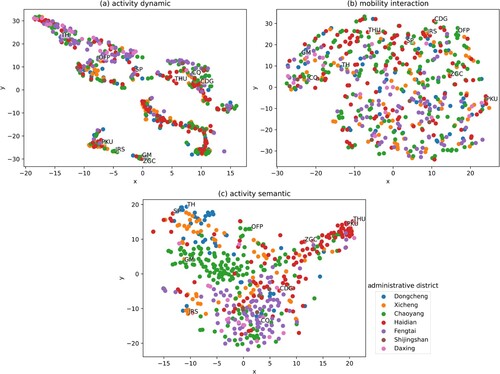
Figure 4. The change of average values and min-max intervals of fuzzy partition coefficient and silhouette score with the increases of cluster number K.
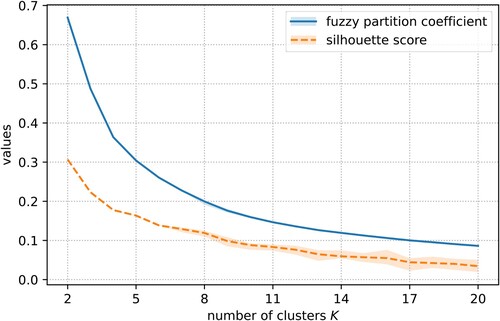
Figure 5. The Pearson’s correlation coefficients between the proportions of urban functions and land use types. *, **, *** denote p < 0.1, 0.01, 0.001, respectively.
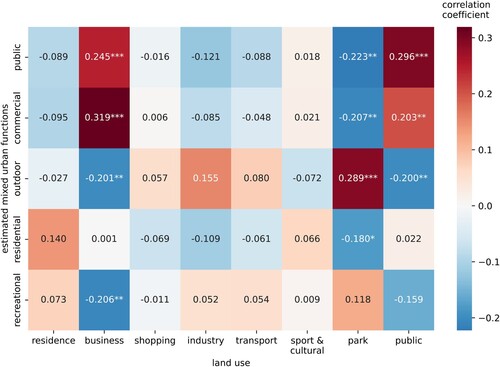
Figure 6. The spatial distribution of mixed urban functions. (a–e) the proportions of five urban functions, (a) public, (b) commercial, (c) outdoor, (d) residential, (e) recreational. (f) the dominant urban function in each zone.
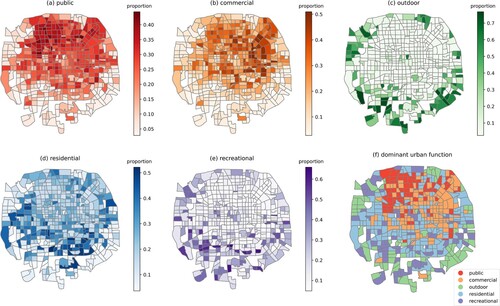
Data availability statement
The Weibo tweet data can be obtained from https://open.weibo.com/. The EULUC-China map data can be obtained from http://data.ess.tsinghua.edu.cn/. The Didi taxi OD data are available from the corresponding author upon reasonable request.