Figures & data
Figure 2. The indicators applied in the HAS map: (a) crevasses, (b) blue ice, (c) slope, (d) wind, (e) strain rate, (f) max buttressing, (g) snow accumulation, (h) ice velocity and (i) air temperature.
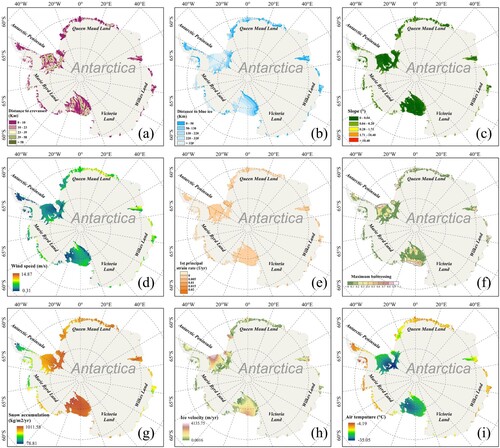
Table 1. Summary of the indicators used in our study.
Table 2. Tolerance and VIF values in multicollinearity diagnosis test for the selected indicators.
Table 3. Three statistical metrics of the machine learning model.
Figure 6. The final HAS map by RF model. We divide Antarctica ice shelves into seven regions, which are labeled and delimited by line segments in dark blue. For each region, we compute area percentages of five HAS classes (pie charts). Yellow represents the testing dataset, green represents the training dataset. Triangle represents the research station sites, and circle represents the human activity records.
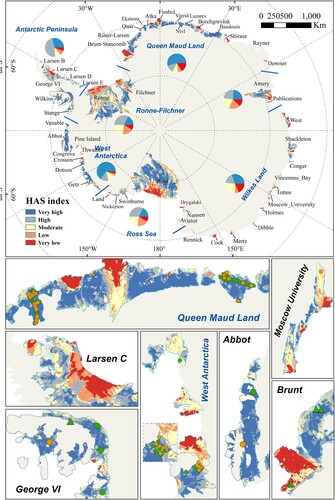
Table 4. Mean values of the selected indicators for the five HAS classes.
Figure 8. Crevasse and blue ice areas (a), HAS index (b), ice velocity (c), maximum buttressing (d) and strain rate (e) of the Ross ice shelf.
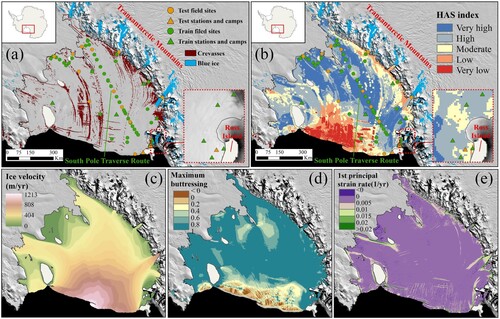
Figure 9. Crevasse and blue ice areas (a), HAS index (b), ice velocity (c), maximum buttressing (d) and strain rate (e) of the Ronne-Filchner ice shelf.
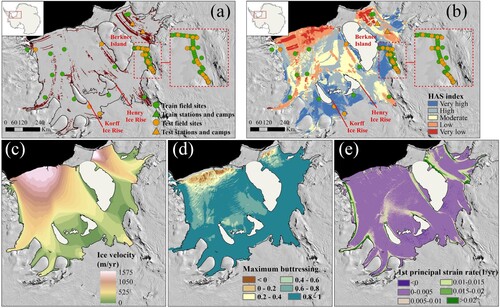
Figure 10. Crevasse and blue ice areas (a), HAS index (b), ice velocity (c), maximum buttressing (d) and strain rate (e) of the Amery ice shelf.
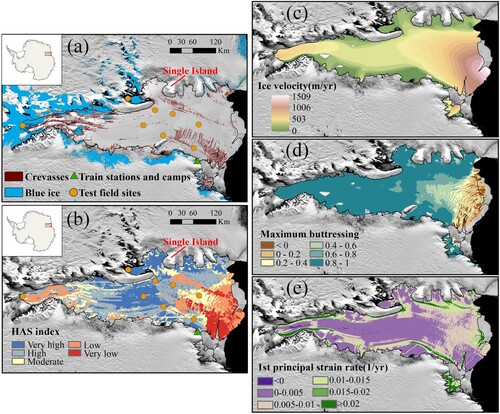
Data availability statement
Data are openly available in a public repository. Antarctic coastline and groundling data used in this study are from National Ice Center (https://usicecenter.gov/Resources/AntarcticShelf). The ERA5 reanalysis data were downloaded from the website (https://cds.climate.copernicus.eu/). The Antarctic ice velocity data were downloaded from the website (https://nsidc.org/data/nsidc-0484/versions/2). The MODIS Mosaic of Antarctica data were downloaded from the website (https://nsidc.org/data/nsidc-0730/versions/1). The fracture location map of Lai et al. (Citation2020) is available at https://doi.org/10.15784/601335. The snow accumulation data were downloaded from the website (https://legacy.bas.ac.uk/bas_research/data/online_resources/snow_accumulation/index.php). The ICESat-2 DEM data were downloaded from the website (https://data.tpdc.ac.cn/en/disallow/9427069c-117e-4ff8-96e0-4b18eb7782cb/). Sentinel-1 imagery is available in Google Earth Engine (https://earthengine.google.com/).