Figures & data
Figure 1. The visualisation results of the training dataset by Labelme annotation tool, presenting original images (a, c) and labelled images (b, d) of certain samples.
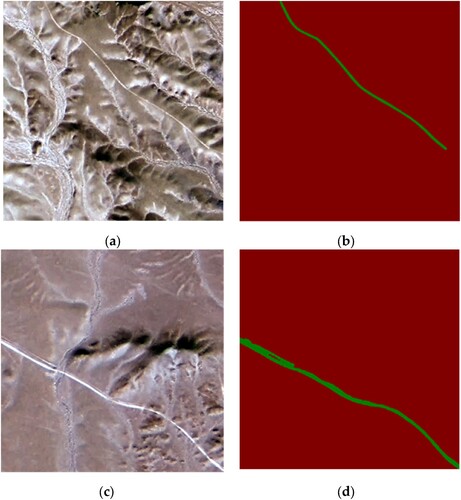
Figure 2. The DeepLabv3 + network backbone structure is usinged in both semi-supervised (UniMatch) and fully supervised models.
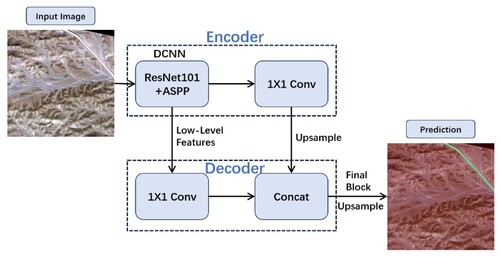
Figure 3. Architecture of fully supervised framework (a) and semi-supervised framework (UniMatch) (b).
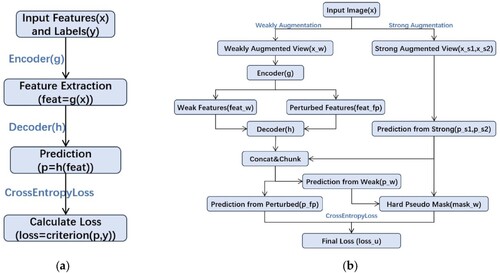
Figure 4. Numerical variation diagram of loss values in the supervised natural road training process (a) and semi-supervised natural road training process (b).
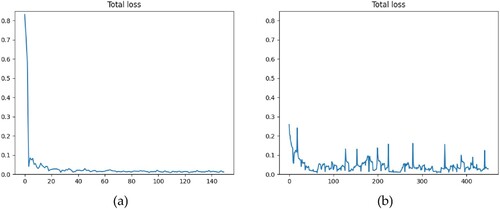
Figure 5. Numerical variation diagram of IOU and MIOU values in the supervised resnet101 -based DeepLabv3 training process (a,c) and semi-supervised UniMatch training process with 1008 unlabelled images (b,d).
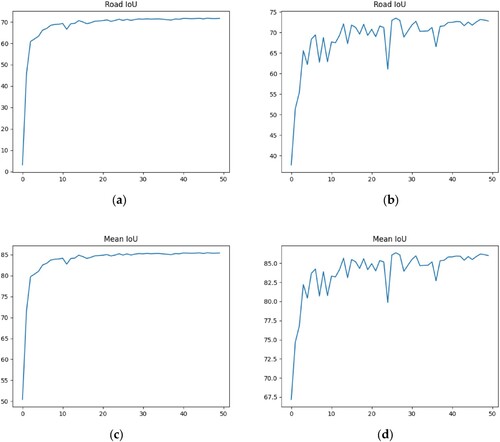
Figure 6. Several sample recognition result images were obtained using the training model of UniMatch semi-supervised network (a, b).
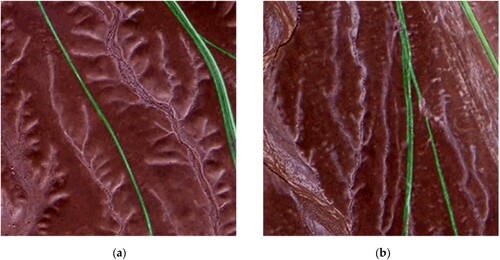
Table 1. Best evaluation indexes of generated model files by supervised and UniMatch semi-supervised network.
Data availability statement
Data and model have been made available on the following GitHub link: https://github.com/wm-jessica/roadseg-back-up.