Abstract
We examine whether barely failing one or more state-mandated high school exit examinations in Massachusetts affects the probability that students enroll in college. We extend the exit examination literature in two ways. First, we explore longer term effects of failing these tests. We find that barely failing an exit examination, for students on the margin of passing, reduces the probability of college attendance several years after the test. Second, we explore potential interactions that arise because students must pass exit examinations in both mathematics and English language arts in order to graduate from high school. We adopt a variety of regression-discontinuity approaches to address situations where multiple variables assign individuals to a range of treatments; some of these approaches enable us to examine whether the effect of barely failing one examination depends on student performance on the other. We document the range of causal effects estimated by each approach. We argue that each approach presents opportunities and limitations for making causal inferences in such situations and that the choice of approach should match the question of interest.
ACKNOWLEDGMENTS
The authors thank Carrie Conaway, the Associate Commissioner for Planning, Research, and Delivery Systems at the Massachusetts Department of Elementary and Secondary Education, for providing the data and for answering many questions about data collection procedures.
Notes
Many states, including Massachusetts, explicitly attempt to limit this barrier by allowing students multiple retest opportunities. For example, some Massachusetts students retake the test more than six times. Nonetheless, some students still cannot meet the passing standard.
They may judge that the extra time spent is not worthwhile; this may be particularly true for students whose scores are very far away from the passing cutoff.
Note that the question of how failing an exit examination affects students is substantively different from the question of how imposing an exit-examination policy affects students. We focus on the first.
Berk and de Leeuw (Citation1999) proposed and adopted a similar approach in their analysis of California's corrections system.
Less than 1% of all students, including those with serious special educational needs, may satisfy high school graduation requirements without taking the MCAS exit examinations. We exclude these students from our analysis.
Results that examine the effect of barely passing one or more exit examinations on college-going within 2 years of the cohort's graduation are available from the first author on request.
The cut scores differ by subject and year. For example, students had to earn 21 points to pass the mathematics examination in 2004 but only 19 points in 2005 and 20 points in 2006. The cut scores in ELA were 39, 38, and 35 points, respectively, across the 3 years. For more information on MCAS scoring and scaling, see the MCAS Technical Reports (Massachusetts Department of Education, Citation2002, Citation2005).
Note that, for completeness, we could also be interested in estimating the effect of passing math and failing ELA, compared to passing ELA and failing math, for students near the joint cutoff (C vs. B). This is not a substantively interesting comparison in our example.
Note that we have adopted to use a linear probability, rather than a logistic or probit, specification of the hypothesized relationship between our outcome—a dichotomous indicator of college attendance—and predictors. As noted by Angrist and Pischke (Citation2008), in large samples, the linear probability specification provides unbiased (consistent) estimates of the underlying trends, while simplifying interpretation enormously. In addition, we use local linear-regression analysis—based only on observations within a narrow bandwidth—and so the linear specification of our statistical models is even more credible (as all trends become increasingly linear, locally, as the ranges of predictors are limited). As a result, when we replicate our analyses using the alternative logistic specification, our results are unchanged and almost identical to those we cite in the article. For example, after replicating our analysis under a logistic specification, we find that barely passing the mathematics examination increases the probability of college enrollment by 2.8 percentage points for prototypical students at the cutoff; the analogous effect in ELA in 4.6 percentage points. These correspond to the estimates of 2.8 percentage points (mathematics) and 4.5 percentage points (ELA) presented in.
We carry out the cross-validation procedure described by Imbens and Lemieux (Citation2008) to determine an optimal bandwidth separately for each subject. In all cases, we find an optimal bandwidth of 2 score points.
In other words, we include (MATHC)2, (MATHC)3, (MATHC)4, (MATHC)5, (ELAC)2, (ELAC)3, (ELAC)4, (ELAC)5, (MATHCxELAC)2 , (MATHCxELAC)3 , (MATHCxELAC)4 , and (MATHCxELAC)5. We test and reject that sixth-order polynomials contribute meaningfully to the prediction.
For example, for a student who fails both tests and for whom MATHC = –3 and ELAC = –5, we use only observations for which MATHC < –3 and ELAC < –5. By contrast, for students who pass both tests and for whom MATHC = 10 and ELAC = 15, we use only observations for which MATHC ≥ 10 and ELAC ≥ 15.
Because data are less dense in the tails of the distribution, including observations that fall far from the cut score in the bandwidth estimation may lead us to select a larger bandwidth than is necessary. As a result, Imbens and Lemieux (2008) recommended deleting observations that fall beyond a certain quantile (δ) on either side of, and most remote from, the discontinuity before implementing the cross-validation procedure just described. Here, we set δ to 25% because the minimum passing scores fall within the tails of the joint distribution of the values of the forcing variables. We conduct this trimming process separately at each value of the forcing variables. In other words, at each value of MATHC , we determine the 25th percentile of ELA scores for observations below the ELA cut score and the 75th percentile for observations above the ELA cut score. We follow a similar process, estimating relevant quantiles of mathematics score at each value of ELAC . Then we exclude simultaneously all observations with MATHC or ELAC scores more extreme than either of these sample quantiles, on either side of the cut score.
Again, we obtain similar results if we change our outcome to student college enrollments within 2 years of their cohort's high school graduation. Thus, it does not appear that the effect of exit examination performance on college-going is simply a matter of delaying college entry.
Table 2 Estimated causal effects of barely passing an exit examination on college enrollment for students on the margin of passing, separately in mathematics and English Language Arts (ELA), by their performance category on the other test, from the frontier RD model in (3) and the response-surface RD model in (4)
Figure 2 Smoothed nonparametric relationship (bandwidth = 2) between the fitted probability of attending college and scores on the mathematics (left panel) and English Language Arts (ELA; right panel) high school exit examinations, with the sample mean probabilities of attending college overlaid. Note. MCAS = Massachusetts Comprehensive Assessment System (color figure available online).
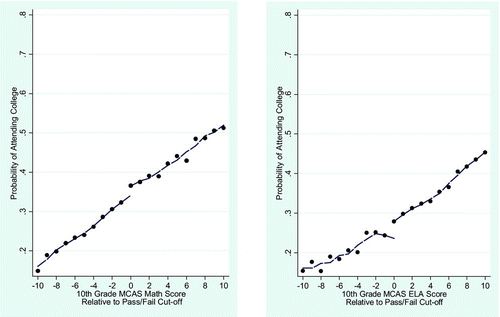
We could construct an analogous plot showing the effect of barely passing the ELA examination by students’ mathematics test score.
Figure 3 Graphical representation of the estimated causal effect of just passing the mathematics exit examination on college enrollment for students at the mathematics cut score, by English Language Arts (ELA) score, with corresponding 95% confidence intervals. Note. MCAS = Massachusetts Comprehensive Assessment System.
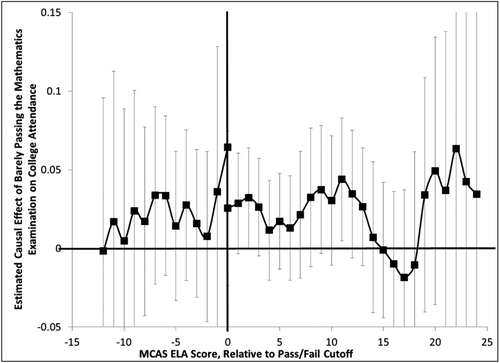
The effect of passing both tests (1), passing ELA for students who pass mathematics (2) and who fail mathematics (3), and passing mathematics for students who fail ELA (4) and pass ELA (5).
Note that we cannot include a cubic term in models where we limit ourselves to a bandwidth of 2 points.