Abstract
Abstract: Community colleges play a vital role in higher education, enrolling more than one in every three postsecondary students. While their market share has grown over the past 50 years, students’ success rates remain low. Consequently, community college stakeholders are searching with mounting urgency for strategies that increase rates of success. We evaluate the effects of one such strategy, learning communities, from a randomized trial of over 1,500 students at a large urban college in the City University of New York (CUNY) system. We find that the program's positive effects on short-term academic progress (credit accumulation) are maintained seven years after random assignment. We find some limited evidence that the program positively affected graduation rates, particularly for those students without remedial English needs, over this time period. While there is not clear evidence that the program improved economic outcomes, this article concludes by offering sobering reflections on trying to detect the effects of higher education interventions on future earnings.
Notes
The first small-scale random assignment study of LCs appears to be by Goldberg and Finkelstein (Citation2002), but it included only 25 students.
The majority of information presented in this section is adapted from Scrivener et al. (Citation2008).
Students whose scores placed them in ESL were not included in the study, as they were eligible for the college's ESL LCs program.
During the first semester of program operations, KCC's LCs program was open only to students between ages 18 and 34 who reported household income below 250% of the federal poverty level. In subsequent semesters, the income criterion was removed, having been deemed unnecessary because such a large proportion of KCC students are from low- or moderate-income families, and 17-year-olds were admitted to the program with parental consent. Age limits were implemented by funder request.
For any semester that a student attended only an institution that does not submit to the Clearinghouse, the student is treated as not enrolled in our analyses.
These records cover about 90% of employment, but they do not capture certain types of jobs, including self-employment, federal government employment, military personnel, informal jobs, and out-of-state jobs.
The research sample, which consists of students who met program eligibility criteria and agreed to participate, may not be representative of the broader KCC student body or the eligible population at KCC. For example, according to IPEDS 2003 fall cohort data, male participants are slightly overrepresented in the research sample (45.4% of the research sample vs. 40.7% of the student body). There is a smaller range of ages in the research sample than the student body because of the eligibility criteria. The research sample also has a higher proportion of Black and Hispanic students than the student body (58.1% of the research sample vs. 48.7% of the student body). In addition, the research sample may look different from the student body on unobservable characteristics.
These were “risk characteristics associated with students’ likelihood of leaving postsecondary education without attaining a credential” described by Horn, Berger, and Carroll (Citation2004). Further information on our proxies of these variables can be found in the sensitivity analysis section.
For a detailed discussion of the implementation research conducted for this program, see Scrivener et al. (Citation2008).
Because there were challenges in managing registration and predicting how many students would test into each level of English, class size varied from six to 25 students, with an average of around 17.
In addition to the four outcomes described here, many other outcomes of interest were explored, some of which are described in the text next. To reduce the multiple hypothesis testing problem (Schochet, Citation2008), two outcomes were prespecified in each outcome domain (academics and employment).
Note that in a previous MDRC report, Sommo, Mayer, Rudd, and Cullinan (Citation2012) presented 6-year graduation impact estimates that had a p value just below.10. Results presented here show a 6-year graduation impact estimate that has a p value of.104, slightly above 10. From a research perspective, the difference is inconsequential. The p value changed because of new information and improved understanding regarding the 6-year degree status of two sample members, including updated data from the Clearinghouse on one of the students.
Table 3. Academic outcomes, Years 1–7
National Student Clearinghouse data enables us to examine colleges beyond CUNY. However, we focus on CUNY colleges in this section because it allows us to break apart enrollment during the main sessions (fall and spring) and the intersessions (winter and summer).
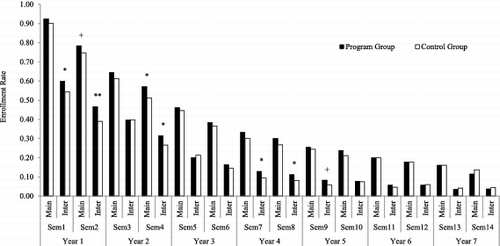
Using National Student Clearinghouse data enrollment at any college covered by the Clearinghouse database was also examined by semester (the Clearinghouse data does not allow a clean breakdown by sessions). During the first 2 years after random assignment, enrollment rates outside of CUNY were low (less than 5%), so enrollment in CUNY colleges (i.e., what is shown in ) is similar to enrollment at any college. During the 3rd through 7th years, enrollment rates at any college were between 5 and 11 percentage points higher than enrollment at CUNY colleges alone. This is important to note when considering the magnitude of enrollment rates over time, because underrepresents enrollment at any college. Of importance, program-control group differences in enrollment rate generally were not affected by enrollment outside of CUNY (where they could be estimated), so the estimated program effects shown in are likely about the same as if they could be examined at any college.
Total credits include both college-level credits (which are generally degree applicable) and developmental credits (which do not count toward a degree).
p =.092. The p value increases due to increased variance in the outcome over time.
The estimated effect on developmental credits all occurred during the 1st year of study and was maintained (but did not grow) during subsequent years.
As noted earlier, more than 20% of the full sample were still enrolled, but nearly half of those enrolled had already completed a first degree.
In this data a positive correlation was found between prerandom assignment employment and future employment. Thus, this difference would appear to favor the control group. Sensitivity analyses were conducted controlling for prerandom assignment employment status—the results were substantively the same.
Table 4. Economic outcomes, years 1-7
The information presented in this section is adapted from Sommo et al. (Citation2012).
The group of students who failed one test consisted almost entirely of those who had passed the reading test but failed the writing test. Only 0.3% of the total sample passed the writing test and failed the reading test.
Table 5. Cumulative credits earned at any CUNY college, by English skills assessment at baseline, years 1-7
Similar tables for other outcome measures are available upon request. They are not included here to save space.
See Scrivener et al. (Citation2008) for more information on these data sources.
The six programs include the subsample of developmental education students in the evaluation described in this article.
For simplicity of exposition, clustering is ignored here. Clustering only exacerbates the issues described.
Seven percent of program group members did not enroll at all during the program semester. An additional 8% enrolled but were not coenrolled in LC courses as of the college's add/drop deadline. Nine percent of control group members did not enroll at all during the program semester. Less than 1% of control group members “crossed over,” meaning they enrolled in an LC. In previous analyses on the 2-year effects of KCC's LC program on the same sample presented on here, Richburg-Hayes et al. (Citation2008) used instrumental variables to estimate the local average treatment effect and observed that the instrumental variables estimates were similar in magnitude and direction to the [ITT] estimates.
One alternative would be to use instrumental variables to estimate local average treatment effect, as in Richburg-Hayes et al. (Citation2008). Such analyses require the assumption that the treatment has no effect on students assigned to the program who did not experience the program, referred to as “no-shows” or “never-takers” (Angrist, Imbens, & Rubin, Citation1996; Bloom, Citation1984). In this study, this assumption may not hold because program participation is defined several weeks into the semester at a point when the program may already have affected students’ outcomes. Moreover, our best program participation measure is defined as coenrolling in LC classes; however, the intervention includes services that may have been received by students who did not coenroll (e.g., textbook voucher, counseling). In other words, the “exclusion restriction” may not hold, so the analyses rely on assumptions that may be violated.
In cases where the value of one or more baseline characteristic could not be conclusively determined due to missing student data, values were imputed to the pooled sample mean. A separate set of “missing” dummy indicators were created when a baseline variable was missing. In addition to the baseline characteristics themselves, these missing dummy indicators were also included as covariates in the sensitivity analyses.
The decision of whether the main analyses would include Xi was made prior to examining the results of the impact model to reduce any potential researcher bias.
Following Horn et al. (Citation2004), students were considered to have delayed postsecondary enrollment if they graduated from high school and enrolled at college in different calendar years. An exception was made for 27 students who graduated from high school in August through November and enrolled in college in January through March of the following year. These students were not treated as having delayed postsecondary enrollment.
Students were considered financially independent if any of the following applied: was 24 or older as of random assignment, was married, or had children (excluding spouse).
Recent research on this topic suggests that cluster robust standard errors can be upward biased in individually randomized group treatment trials such as this one. This occurs because students were nonrandomly sorted into LCs after random assignment resulting in the appearance of dependency of observations within clusters, which need not be accounted for in the analyses since it is artificial (Weiss, Lockwood, & McCaffrey, Citation2014).
It is reasonable to use OLS with this study design if the desired inference is with respect to the effect of the specific LCs observed in this study (taught by the specific instructors observed in the study) rather than to a hypothetical superpopulation of LCs from which these particular LCs could have been randomly drawn (Siemer & Joorman, Citation2003b). Technically, Siemer and Joorman (Citation2003b) recommended a constrained fixed effect model that will often produce standard errors that are smaller in magnitude than OLS; however, recent work on this topic by Weiss et al. (2014) suggests that the constrained fixed effects approach can produce standard errors that are downward biased (owing to the nonrandom a sorting of students into clusters), and thus OLS may be a reasonable alternative for those interested in the fixed effects inference.
The standard error of the impact estimate decreased by an average of 10.4% for credit accumulation and 18.0% for degree completion.
With respect to credit accumulation, the two approaches yield nearly identical standard errors and p values, with differences in p values all below.01. With respect to graduation, the p values using ordinary least squares are, on average,.07 smaller than when using cluster robust standard errors. Substantively, this does not change the story. With respect to employment, p values are generally slightly smaller using ordinary least squares, with the largest decrease being.025.