Figures & data
Table 1. List of uncertain parameters in the calibration framework and selected prior probability distributions.
Figure 1. Photos showing the Architecture Studio building (left) and the energy supply system cabinet with the heat pump on the bottom and the buffer tank on the top.
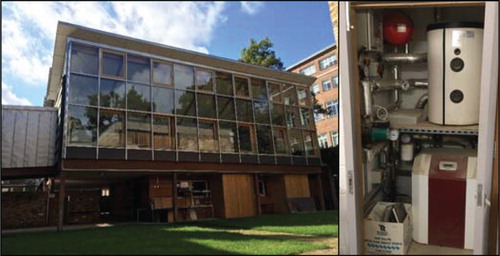
Figure 2. Schematic of the heat pump model in cooling mode with the corresponding input and output parameters. Uncertain parameters selected as calibration parameters are in italic.
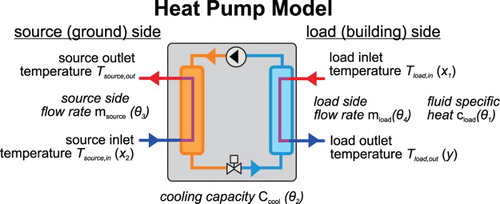
Figure 3. Measured field data and simulated computer data for the three data sets A, B and C showing the different temperature ranges and trends of each data set across the two dimension of load (upper plots) and source inlet temperature (lower plots).
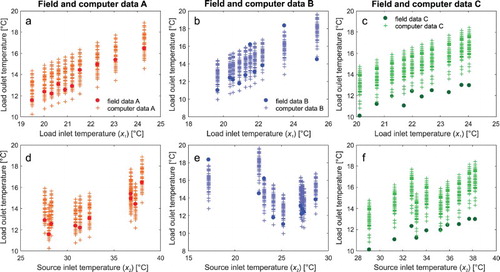
Figure 4. Results for predictive simulations for the emulator (a, d, g), the whole model term (b, e, h) and the model bias function (c, f, i) for data sets A, B and C over the load inlet temperatures (x1).
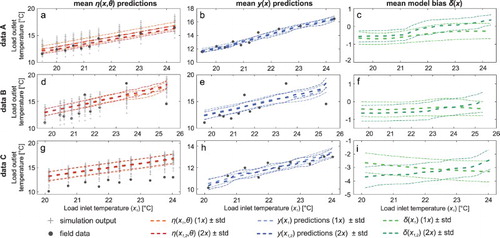
Figure 5. Results for predictive simulations for the emulator (a, d, g), the whole model term (b, e, h) and the model bias function (c, f, i) for data sets A, B and C over the source inlet temperatures (x2).
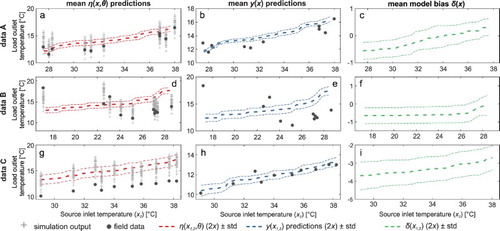
Figure 6. Prior and posterior probability distributions shown as boxplots for the reciprocal of the precision hyper-parameter λb and the correlation hyper-parameters (βb1 refers to x1, i.e. load side flow rate, βb2 refers to x2, i.e. source side flow rate) of the model bias for the three investigated data sets A, B and C. The centre line represents the median of the posteriors, boxes cover the interquartile range and whiskers include approx. 99% of the obtained posterior samples. The dashed line indicates the magnitude of the prior median value. Please note the parameter values do not represent the absolute value of the error term, as they are inferred from the normalized model data.
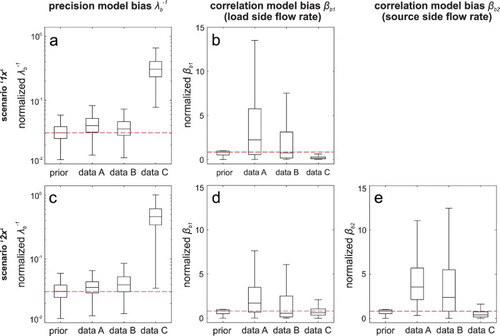
Figure 7. Prior and posterior distribution for the reciprocal of the random error λe and numerical error λen for different data sets on logarithmic scales. The centre line represents the median of the posteriors, boxes cover the interquartile range and whiskers include approx. 99% of the obtained posterior samples. The dashed line indicates the magnitude of the prior median value. Please note the parameter values do not represent the absolute value of the error term, as they are inferred from the normalized model data.
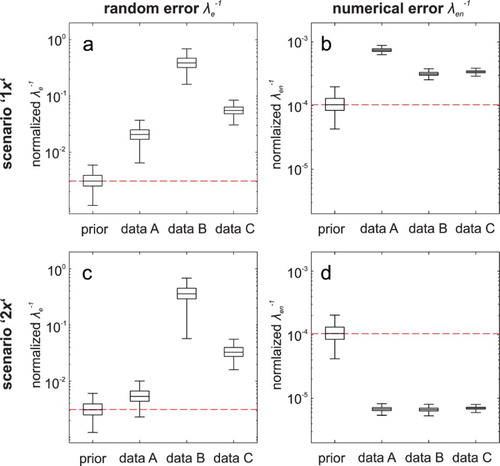
Figure 8. Prior and posterior distributions as boxplots for the four calibration parameters θ for different data sets. The centre line represents the median of the posteriors, boxes cover the interquartile range and whiskers include approx. 99% of the obtained posterior samples. The dashed line indicates the magnitude of the prior mode value.
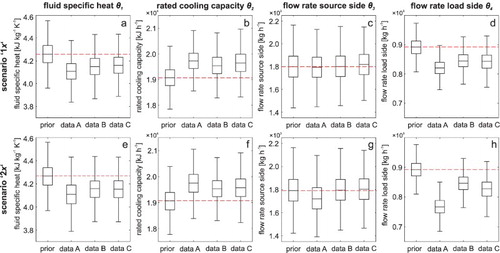
Figure 9. Posterior distributions of θ1, θ2, θ4, λe−1 and βb for data sets A, B and C under scenario ‘1x’ for different priors on λb (GAM(10, 0.03), GAM(10, 0.1), GAM(10, 0.3), GAM(10, 1), GAM(10, 2.5) corresponding to an amount of variation in y caused by the model bias of ∼0.3%, ∼1%, ∼3%, ∼10% and ∼25%. The base case (∼3%) is indicated in bold. Resulting posteriors for θ3 are not shown as this parameter is unidentifiable as well, and does not show any changes.
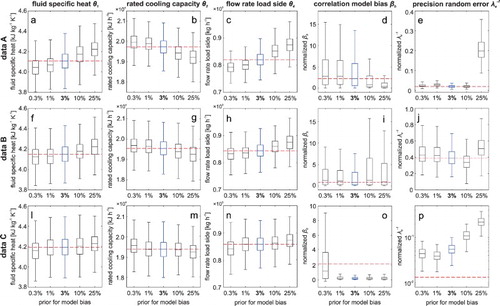
Figure 10. Prior distributions and combined histogram plots of all posterior samples from 199 converged calibration runs with 2000 samples each, inferred from the evaluation of the random data sets. The circles indicate the posterior median values of the individual 199 posterior sample sets, while the vertical solid and dashed lines indicate the posterior medians of data A and B, respectively. Note the logarithmic scale on the x-axes for the inverse of the model bias λb−1, measurement error λe−1 and numerical error λen−1.
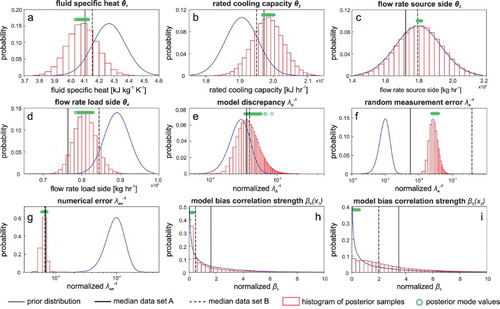