Figures & data
Table 1. Case study cities.
Figure 6. Network typologies for Bay Area. Neighborhood residential streets constitute the highest share, followed by highways. Individual cities reflect similar partitioning.
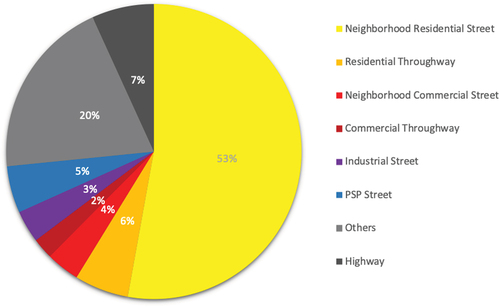
Figure 7. Network typologies for Oakland, San Jose, San Francisco, and Concord. Oakland has the highest percentage of highways, and Concord has the highest percentage of neighborhood residential streets.
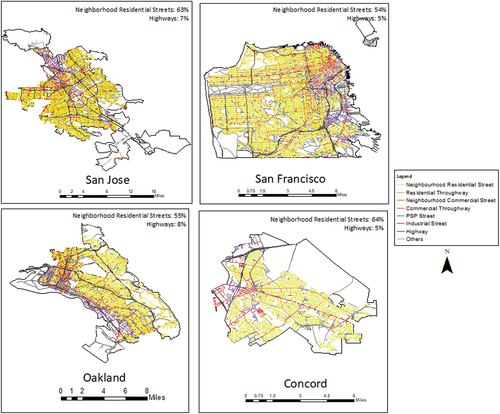
Table 2. Methods used to evaluate indicators.
Figure 8. Theme Neighborhood: Communities of Concern census tracts in the case study cities. Oakland has the highest percentage of population living in these census tracts and Concord the least.
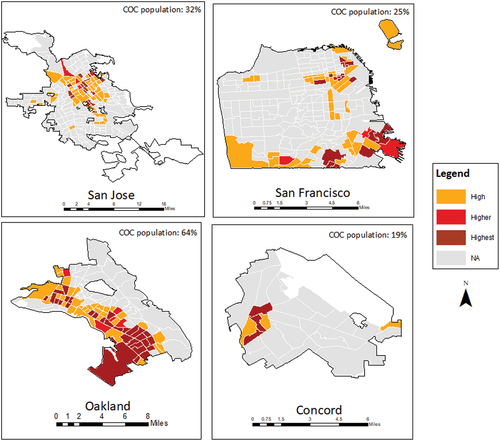
Figure 9. SAEF indicators for San Jose. Each metric is normalized to a scale between 0 and 1. Red and blue represent high and low, respectively. For instance, VHD indicator in the mobility theme is high for SOF and low for SOT
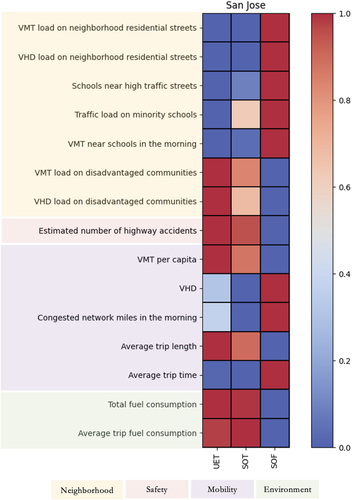
Figure 10. Theme Neighborhood: The figure illustrates the schools (blue circles) affected by high and medium traffic volume. Red links have an ADT greater than 50,000, and yellow links have ADT between 25,000 and 50,000. The number of impacted schools is higher with SOF routing.
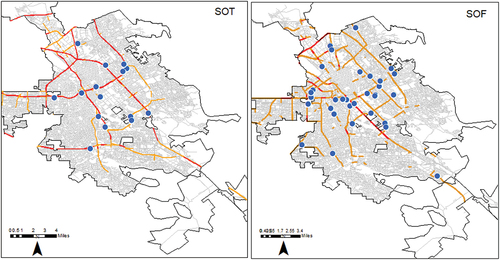
Figure 11. Theme Mobility: The figure shows the difference in VMT for SOT (left) and SOF (right) compared to baseline UET for San Jose. The red or green represents the increase or decrease in VMT, and the thickness represents the magnitude of the difference. Only highways and neighborhood residential streets are shown. It can be seen that SOF has shifted a significant amount of traffic from highways (broad green lines) to residential streets (thin red lines) in an attempt to reduce fuel consumption.
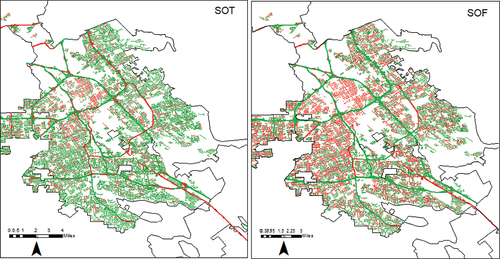
Figure 12. The figure compares SAEF metrics across cities for different route optimizations. The metrics are numbered from 1 to 15 on the chart. They are normalized with respect to the maximum values for a city so that the relative differences can be identified. For example, in San Jose, SOF has the highest values for metrics 1, 2, 3, 4, 5, 10, 11, and 13. In San Francisco, UET has the highest values for metrics 2, 3, 6, 8, 9, 10, 11, 14, and 15. Overall, the comparison reveals that UET performs worse for San Francisco whereas SOF performs worse for all other cities.
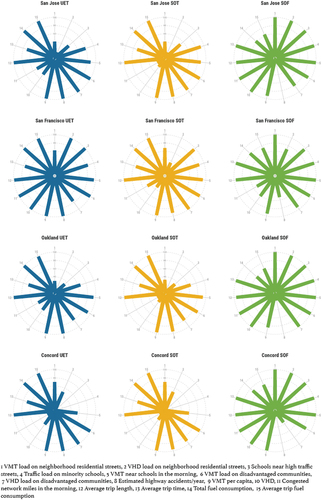
Table A1. SAEF indicator description.
Table A2. Functional road classes.
Table A3. Network typologies for Bay Area.
Table A4. SPF parameters for California highways.
Table A5. Summary of Results: SAEF for case study cities.