Figures & data
Figure 3. Model Builder scheme used for creating buffer layers and database files with frequency of intersected crimes.

Figure 4. Spatial distribution of gambling sites and selected offences (property damage, violation of noise ordinance and thefts) in the city of Pardubice in 2014.
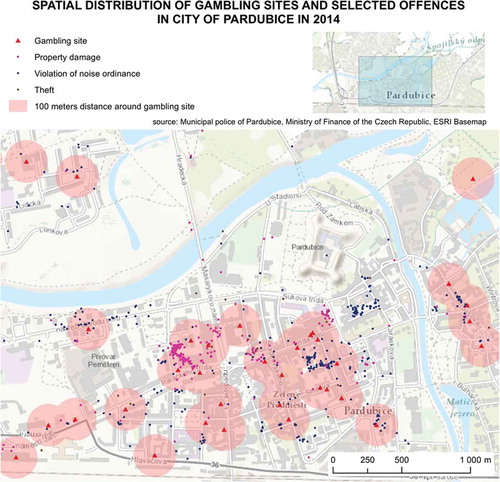
Figure 5. Correlation between value of distance for buffer and relative number of crimes for selected offence types.
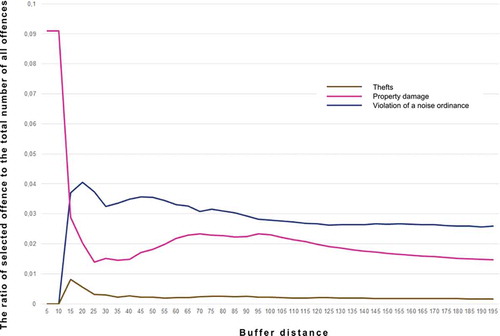
Figure 7. Heat map created using kernel density estimation depicting clusters of offences caused by cyclists.
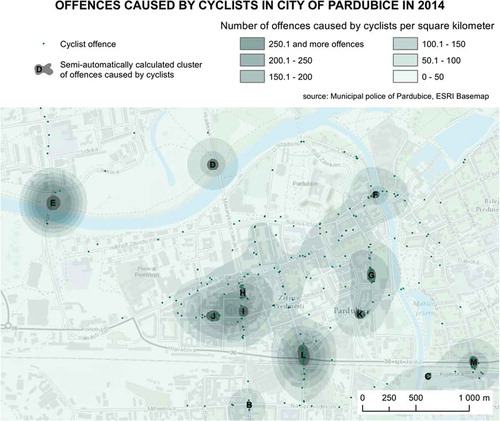
Table 1. List of identified clusters with description of most frequent offence types and proposal of possible solutions.