Figures & data
Figure 1. Chunked storage and unified storage (a) chunked storage with multilayer array indexing, (b) unified storage with no extra indexes
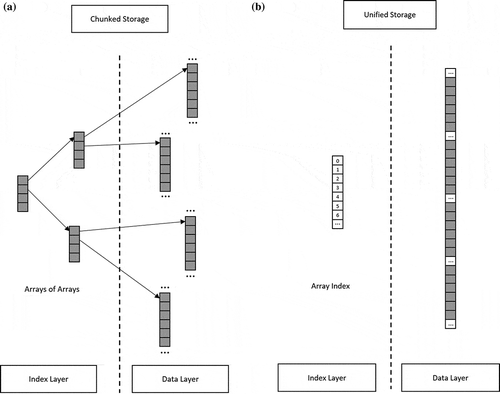
Table 1. Spatiotemporal queries
Table 2. Pre-processing data (from NetCDF to CSV)
Table 3. Data pre-processing & uploading time and data size in different containers