Figures & data
Figure 1. The flowchart of the proposed representation learning framework for extracting hierarchical intra-urban spatial structure.
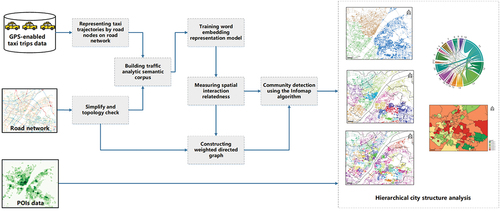
Figure 2. An example of mapping a raw taxi route to intersection nodes sequence along the road network.
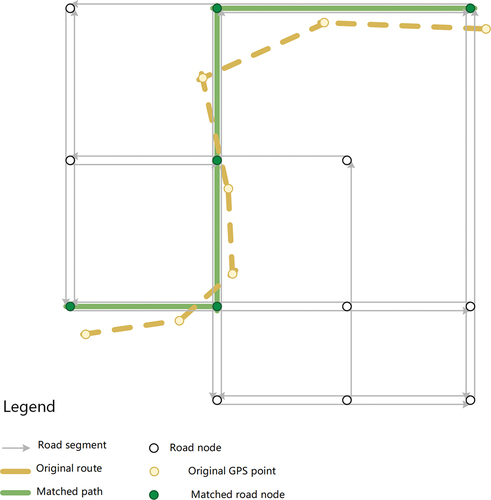
Figure 3. Our study area in Wuhan, which covers the downtown area of this city (within the third ring road). (a) Administrative districts of Wuhan city; (b) Satellite remote sensing image of the study area. The bottom images show examples of diverse landscapes: (1) industrial area, (2) residential area, (3) commercial area and (4) educational area.
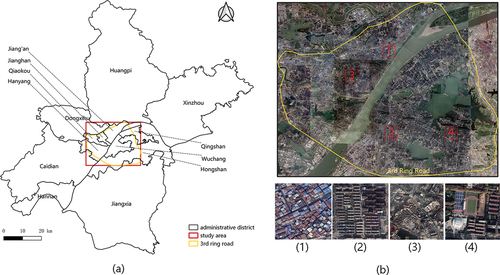
Figure 5. (a) kernel density estimation of pois (unit: count per km2); (b) spatial distribution of POIs near Jianghan road, a famous commercial area within the downtown of Wuhan. Note that different colours indicate types of POIs.
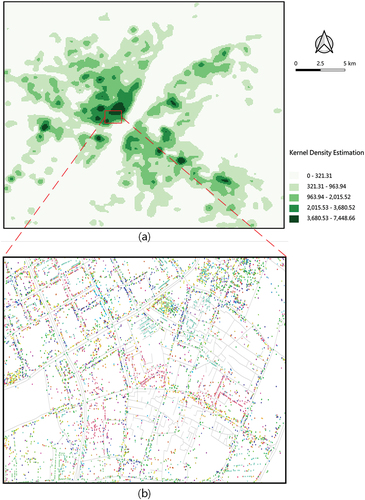
Figure 6. Relationship between the average similarity of node embeddings and the distance between two nodes.
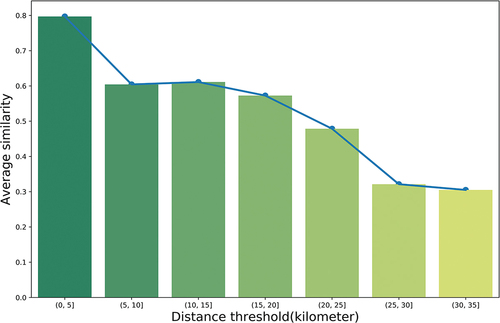
Figure 7. An example of embedding vectors in a road network graph: (a) road networks; (b) embedding vectors of road nodes; (c) spatial distribution of cosine similarities.
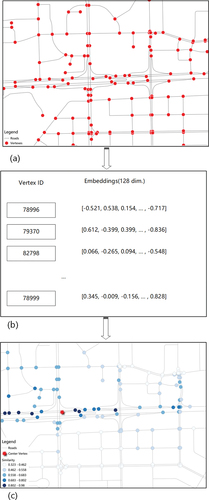
Figure 8. The spatial distribution of hierarchical communities. Note that the solid black lines indicate the administrative boundaries. (a) top-level communities; (b) second-level communities; (c) third-level communities; (d) and (e) indicate the second-level divisions and third-level divisions of Jianghan district.
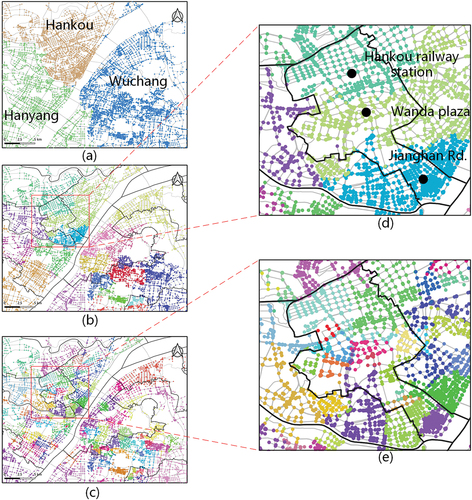
Table 1. Comparison of different communities.
Figure 9. The chord diagram of traffic flows using origins and destinations of taxi movements. noting that the numbers in the left diagram and right map is consistent and indicate the identifier of the sub-regions. (a) the chord diagram; (b) the spatial distribution of sub-regions. the blue lines indicate the sub-regions boundary generated by Thiessen polygon algorithm.
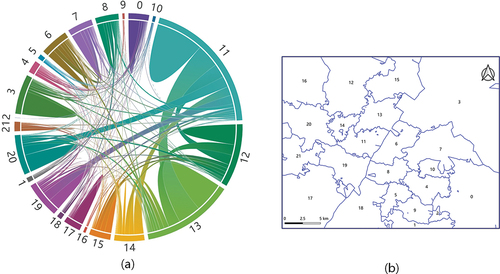
Table 2. Urban functions areas and example locations.
Table 3. The percentage of average frequency of taxi flows between the second-level divisions within the same division of seven days.
Table 4. Indices for mixed land use of the third-level divisions.
Data availability statement
The data and codes that support the findings of this study are available from the corresponding author upon reasonable request.