Figures & data
Table 1. DenseNet + GC + SE network architecture.
Table 2. Physical and mechanical parameters of the sample and loading rate.
Figure 9. Acoustic emission waveforms at different stages. (a) Stage one (b) stage two (c) stage three (d) stage four (e) stage five (f) stage six.
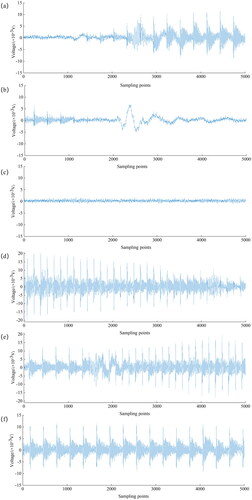
Figure 10. Waveform after wavelet transform of acoustic emission. (a) Stage one (b) stage two (c) stage three (d) stage four (e) stage five (f) stage six.
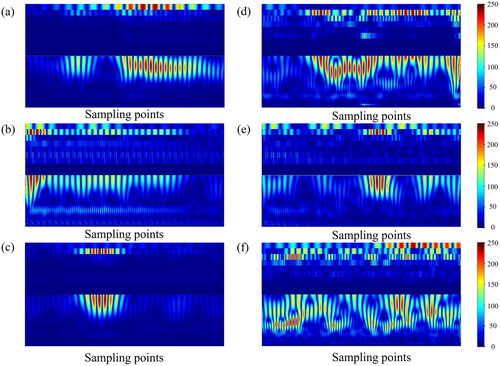
Table 3. YOLO model performance comparison table.
Table 4. Model training parameters.
Table 5. Validation set training results.
Figure 15. The utility of group convolution in characterizing the diversity of acoustic emission features.
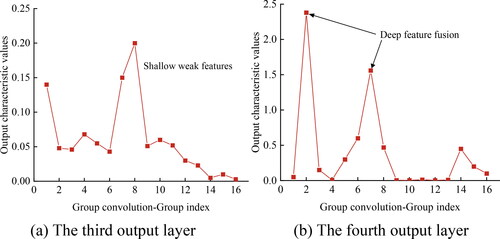
Figure 16. The utility of the SE module – representing the importance of acoustic emission features.
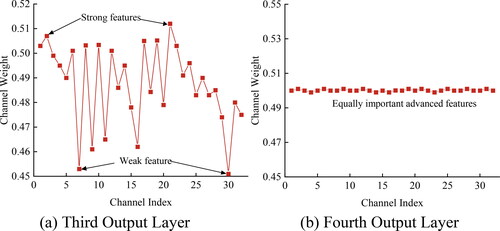
Table 6. Comparison of different 3D network performances.
Data availability statement
All the data, models or code generated or used in the present study are available from the corresponding author by request.