Figures & data
Table 1. Model hyperparameter setting.
Table 2. The characteristics of acceptable results for RPE, RMSE, MAE, and NSE.
Table 3. Summary of the training dataset (count = 460).
Table 4. Summary of the testing dataset (count = 92).
Table 5. Optimal PSO-ML hyperparameters for different lead times in rainfall-runoff simulation.
Table 6. Optimal PSO-ML hyperparameters for different lead times in EFI-runoff simulation.
Figure 11. Rainfall, EFI, and runoff time series on the held-out testing samples with different lead times.
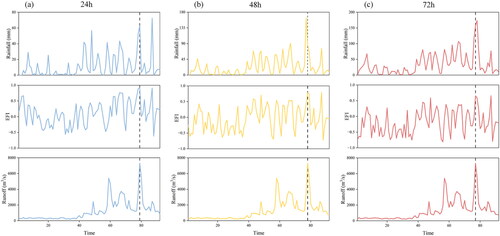
Figure 12. Multiple model prediction results on the held-out testing samples obtained by two inputs with different lead times.
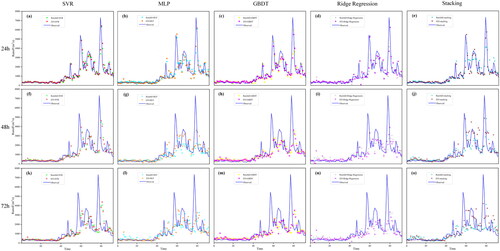
Table 7. Evaluation indices comparison of two inputs.
Data availability statement
Data will be made available on request.