Figures & data
Figure 1. Process example of resource allocation for X nodes: (a) the bi-partite graph, (b) the X and Y projections, and (c) the number of common neighbours in Y and X – Source Zhou et al. (Citation2007)
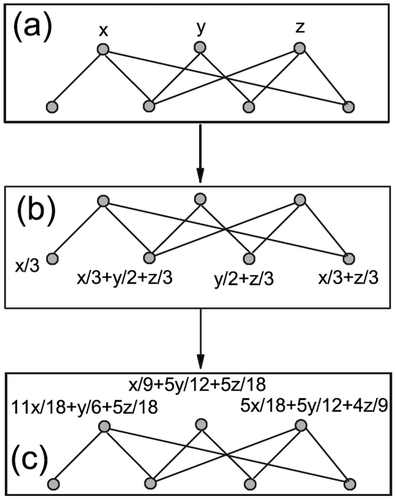
Figure 2. Room with IoT infrastructure and possible service components – Source Cisco Systems (Citation2009)
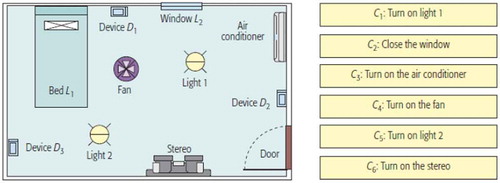
Figure 4. Bi-partite graph representing IoT devices and service components of a smart home environment
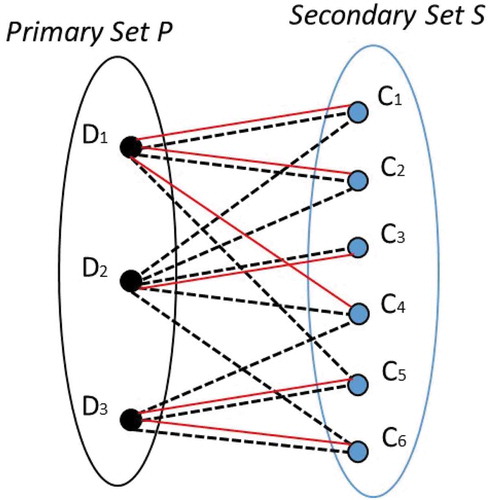
Figure 6. Comparison of community detection algorithms – Source Papadopoulos et al. (Citation2011)
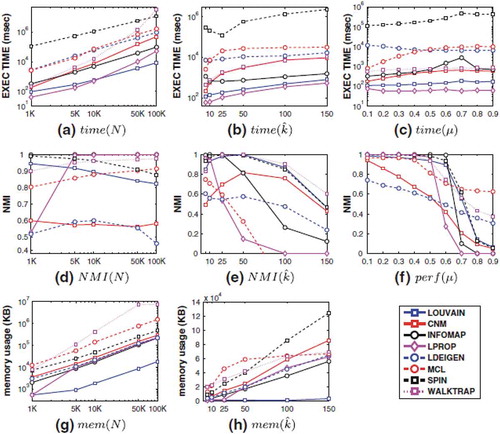
Table 1. Steps to allocate dmin according to the current velocities of IoMT devices
Table 2. Steps to create a bi-partite graph
Table 3. Steps to find communities in IoMT networks
Table 4. Example of IoMT data collected by a smart car
Table 5. Overview of the community detection results
Data availability statement
The data that support the findings of this study are openly available in Next Generation Simulation (NGSIM) Vehicle Trajectories and Supporting Data (opendatanetwork.com) at https://www.opendatanetwork.com/dataset/data.transportation.gov/.