Figures & data
Figure 1. Schematic comparison of Pixel-Based Classification (PBC) and Object-Based Classification (OBC). Top Row: on VHR, OBC more accurately detects vegetation, while PBC comprises mix pixels. Bottom Row: Spatial resolution and size of the pixels define either OBC or PBC can be more effective. Regarding MR, OBC is inefficient as a pixel contains more than one object, while PBC still detects vegetation (Source for the background satellite image: Google Earth Engine)
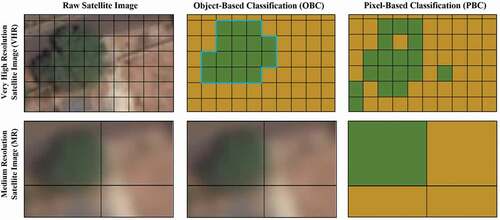
Figure 2. Random forest algorithm’s schematic function in image classification. The classifier consists of a collection of individual trees. Each tree independently offers a class prediction. The class with the most votes becomes the model prediction for image classification
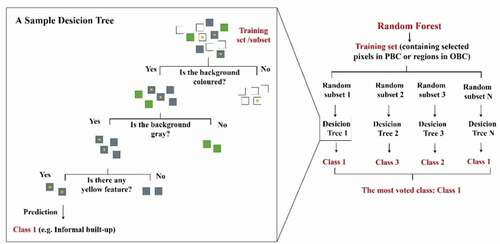
Figure 3. Study areas. Small rectangles numbered from 1–6 represent 2.4 km2 sample areas for the visualisation and the magnification of the results
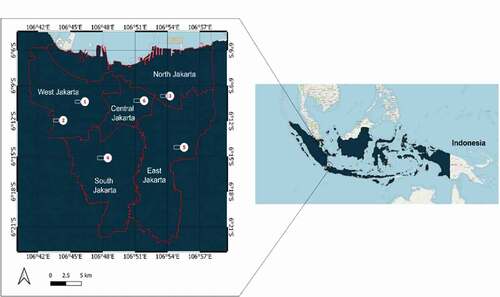
Figure 4. Informal settlements of Jakarta adapted from a map produced in 2015 (The World Bank Citation2015)
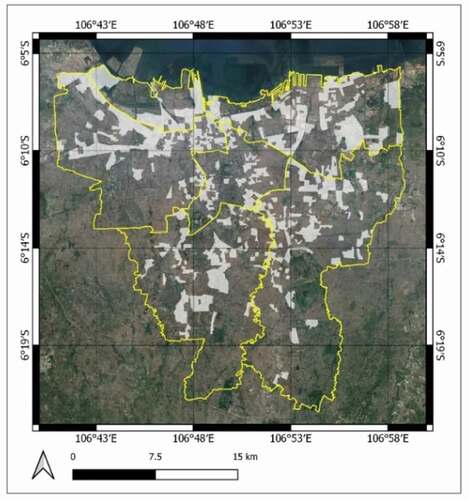
Figure 5. Overview of the procedure followed to detect informal settlements using medium resolution satellite images (MR), OpenStreetMap (OSM) and random forest machine learning algorithm (RF)
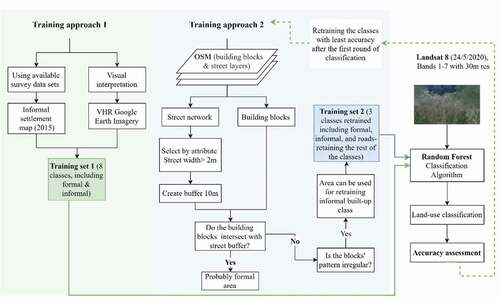
Table 1. Proposed classes for the classification of Landsat 8 in Jakarta
Figure 6. Left: Street structure in informal settlements in Jakarta. Numbers 1–4 represent the entry points to the informal settlements with no car accessibility (Source: Hidayati et al., Citation2020), Right: Urban fabric in informal settlements (Source: Alzamil, Citation2018)
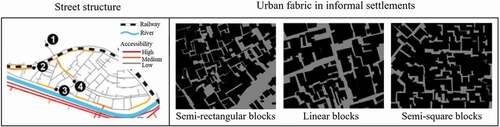
Figure 7. Using OpenStreetMap (OSM) for developing the training set for the informal built-up class. The blue areas represent the building with no access to a street or access to narrow streets that have been used to select pixels for informal settlements (Source for data layers: OpenStreetMap)

Table 2. Confusion Matrix of RF for the first round of classification. Least accuracy and precision relate to formal built-up in which mainly roads and informal built-up have been wrongly classified as formal
Figure 9. Second round of land-use and land cover classification on Landsat 8 using OSM for developing the training set
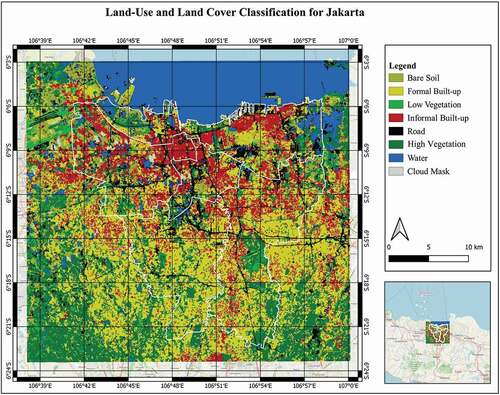
Figure 10. Informal (in red) and formal (in yellow) settlements detected in Jakarta. 1. Classification by RF machine learning algorithm through the traditional method of training. 2. Classification by RF which takes advantage of OSM for developing the training set
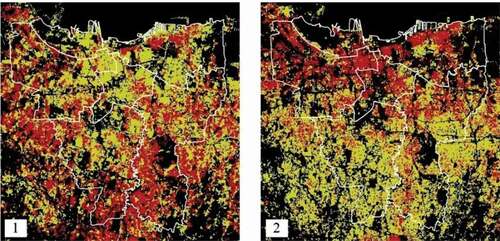
Table 3. Confusion Matrix of RF for the second round of classification
Figure 11. Comparison between the roads detected in the first and second round of classification (roads are mapped as black lines). Roads in the first round of classification are not continuous and represent some noise. Unlike the first-round results, street lines in the second round are mainly unbroken and demonstrate a high level of precision that is represented in
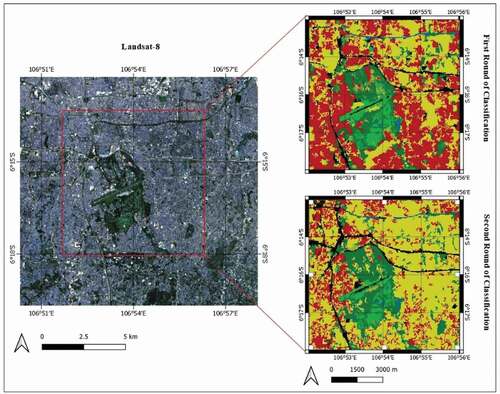
Data availability statement
Open data used for this study is freely available to public through the Copernicus Open Access Hub (https://scihub.copernicus.eu/) and OpenStreetMap Indonesia (https://openstreetmap.id/en/dki-jakarta/).