ABSTRACT
Modelling source water quality in drinking water treatment systems could be useful for anticipating changes in specific raw water quality parameters. Those changes entail adjustments in drinking water treatment plant (DWTP) operations. Artificial intelligence (AI) has been used for modelling water quality for different purposes and has yielded reliable results. However, there has not yet been wide investigation of raw water quality modelling for treatment purposes using AI. For the first time, in this critical review, we analyzed AI models founded on machine learning techniques that are used for surface water quality modelling and which could be applied in the domain of source water treatment. In a novel approach, we convened an expert panel that helped us define the appropriate criteria for use in the selection of the papers for review. We analysed the selected papers according to several criteria, including the feasibility of input data generation, the modelled data applicability and the benefits and limitations. We evaluated whether the selected models could be applied to forecast raw water quality as decision support systems (DSS) in drinking water treatment. The highest rated were turbidity hourly models based on Support Vector Machines (SVM), as well as daily turbidity and pH models based on Artificial Neural Networks (ANN). We found there is a shortage of models used to specifically estimate raw water quality, which could assist in DSS at DWTPs. There should be an increased effort to model raw water quality, especially with AI models using hourly and sub-hourly time step.
GRAPHICAL ABSTRACT
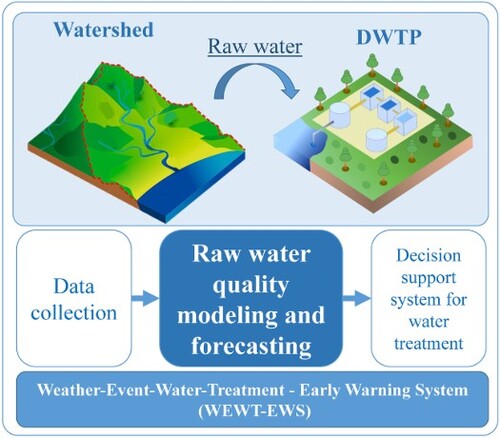
Acknowledgements
The authors acknowledge the funding support by the NSERC (Natural Sciences and Engineering Research Council of Canada) Drinking Water Research Chair of Laval University, whose main partners are the municipalities of Quebec City and Lévis, Avensys Solutions, Agiro and WaterShed Monitoring. Also, the authors would like to acknowledge their gratitude and appreciation to the members of the expert’s panel. The authors are thankful to Blue-Pencil for English proofreading of the paper.
Disclosure statement
No potential conflict of interest was reported by the author(s).
Data availability statement
Data sharing is not applicable to this article as no new data were created or analyzed in this study.
Additional information
Funding
Notes on contributors
Christian Ortiz-Lopez
Christian Ortiz-Lopez is currently a PhD candidate in land use planning and regional development at Université Laval in Quebec, Canada, within the Drinking Water Research Chair. He has a BS degree in civil engineering (2012) at Universidad Católica de Colombia, a BS degree in environmental management (2007) at Universidad Distrital Francisco Jose de Caldas and a MSc degree in hydrosystems (2012) at Pontificia Universidad Javeriana in Colombia. His doctoral research project is related to the development of data-driven models for raw water quality forecasting during rainfall events and decision support tools for drinking water treatment. Other research interests, source water quality and monitoring, machine learning models for source water quality, protection of water sources for supply, optimization of drinking water treatment systems.
Christian Bouchard
Christian Bouchard has a BS degree in engineering (1980) at the École Nationale Supérieure des Arts et Métiers in Paris, a MSc degree in applied sciences in civil engineering (1982) and a PhD in chemical engineering (1990) at the École Polytechnique from Montreal. During his PhD, he was a visiting scholar at the National Research Council of Canada in Ottawa. After working for nearly 2 years at the Quebec Ministry of the Environment in Montreal, he became a professor at Laval University in the Department of Civil Engineering in 1992 where he teaches and does research in the field of environmental engineering. He has worked within the Drinking Water Research Chair at Université Laval since 1997. His research work has largely focused on the development and improvement of processes for water treatment. In particular, for several years he has been studying membrane filtration processes (nanofiltration, ultrafiltration) applied to the production of drinking water, the treatment of wastewater and the recovery of food products.
Manuel Rodriguez
Manuel J. Rodriguez has a BS degree in civil engineering, a MSc degree in land use planning and regional development (ATDR) and a PhD in environmental engineering. He completed postdoctoral fellowships in England and France in the field of drinking water quality. He is a full professor at the Higher School of Land Use Planning and Regional Development (ESAD) at Université Laval, where he teaches in the fields of environment and water. He is a regular member of the Center for Research in Planning and Development (CRAD) and the Center for Water Research (c-Eau) at Université Laval. Professor Rodriguez’s research programme concerns the management of drinking water quality, with an integrated approach from the watershed to the consumer’s tap. He holds the CRSNG Drinking Water Industrial Research Chair at Laval University.