Figures & data
Figure 1. Overview of multilevel RNA-Seq pipeline evaluation approach. Bold arrows, analysis pipeline; dashed gray arrows, tested influences of various analysis stages on the robustness of the resulting functional signature (the latter was measured as correspondence between the functional signatures inferred for cellular responses to natural and artificial media toxicity).
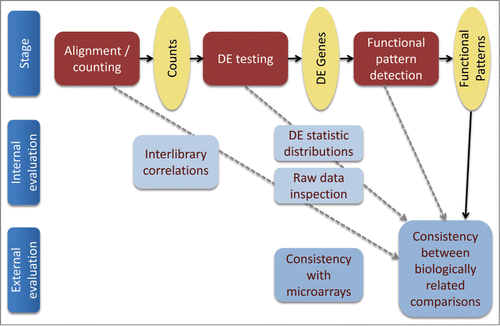
Figure 2. Related biological comparisons used. Naturally toxic medium: ammonia-pretreated corn stover hydrolysate (ACSH); artificially toxic medium: synthetic hydrolysate with added cocktail of toxic compounds discovered in ACSH (“lignotoxins”); control medium: synthetic hydrolysate with lignotoxins omitted. SeeCitation15 for further details.
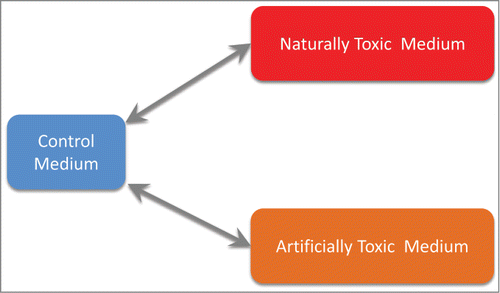
Figure 3. Number of pairwise comparisons a particular combination of read preprocessing and alignment / counting method resulted in the maximal value of Pearson correlation between the genome-wide vectors of counts. (A), winning cases out of all the 210 pairs, irrespective to experimental conditions; (B), winning cases out of 15 interreplicate comparisons. Cyan, BWA-HTSeq pipeline; blue, Bowtie-RSEM pipeline.
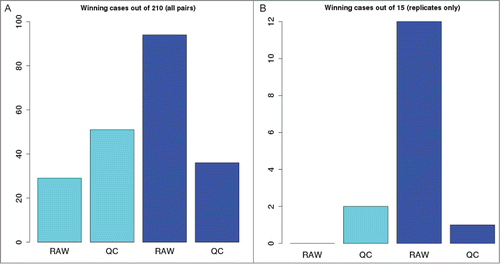
Figure 4. Critical Coefficients computed for genes that are called differentially expressed (FDR < 0.05) in artificially toxic medium at exponential growth phase by 4 DE calling methods. For EBSeq, posterior Probability of Equal Expression was used as a conservative estimate of FDR. (A), EBSeq; (B), DESeq; (C), edgeR; (D), voom / limma. Red, genes with critical coefficient values below 1 (corresponds to 0 in the log-scale applied). Black and red inline numbers are numbers of genes that are called DE by a particular method and show either absence (crt >=1, black) or presence (crt<1, red) of inconsistent sign of the transcript abundance changes between the 2 conditions at individual replicate level.
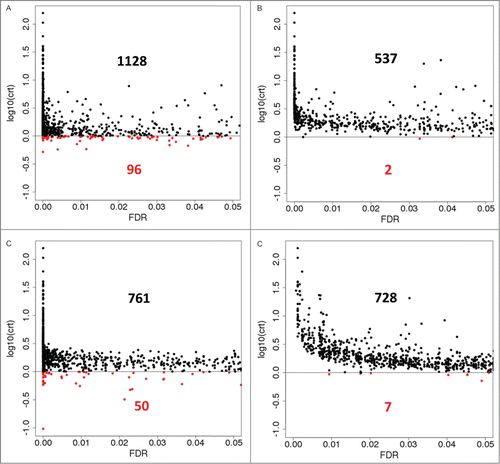
Table 1. Generalized Linear Model of the RNA-Seq - microarray DE results concordance (expressed as RI) as a function of the technical and biological factors combined. Levels of factors reported: QCRAW, no read pre-processing; alignRSEM, RSEM alignment / counting pipeline; normTMM, count normalization using TMM; normUPPER, count normalization using upper quartile; compsynHLT, biological comparison of SynH+LT vs. SynH; timeT3, transitional phase of cellular growth; timeT4, stationary phase of cellular growth; DEEBSeq, EBSeq as the DE calling algorithm; DEedgeR, edgeR as the DE calling algorithm; DEvoom, voom / limma as the DE calling algorithm; critTRUE, critical coefficient of 1.15 applied. Bold, variables retained after forward-backward regression and p-value filtering.
Table 2. Generalized Linear Model of the correlation between vectors of functionality as a function of the technical and biological factors combined. Levels of factors reported: DEDirectionUP, genes upregulated in toxic media; GeneSetTypesPW, species-specific pathways as gene set type; GeneSetTypeTF, regulons as gene set type; GeneSetTypeTransp, Transporters as gene set type; FunSearchTypepiano, Fisher's gene-level p-values summarization as enrichment test; FunSearchTypepianoFOLD, gene-level summarization of fold changes as enrichment test; PreProcessRAW, nor read pre-processing; AlignCountRSEM, Bowtie-RSEM alignment / counting pipeline; NormalizTMM, TMM count normalization; NormalizUPPER, upper quartile count normalization; TimePointT3, transitional phase of cellular growth; TimePointT4, stationary phase of cellular growth; DEMethodEBSeq, EBSeq as the DE calling algorithm; DEMethodedgeR, edgeR as the DE calling algorithm; DEMethodvoom, voom / limma as the DE calling algorithm. Bold, variables retained after forward-backward regression and p-value filtering.
Figure 5. Distributions of correlation coefficients between vectors of -log10(GS-FDR) values computed for ACSH vs. SynH and SynH+LT vs. SynH comparisons for up- and downregulated genes.
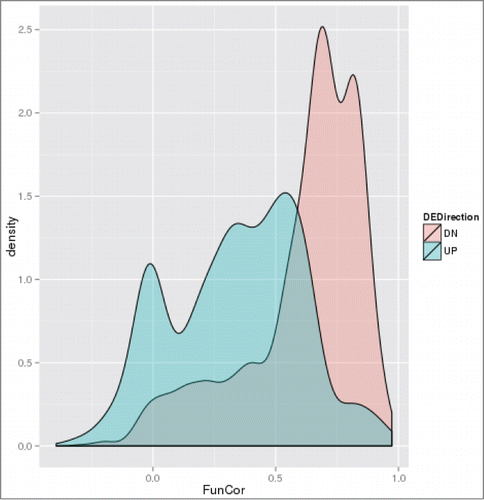
Figure 6. Distributions of correlation coefficients between vectors of -log10(GS-FDR) values computed for ACSH vs. SynH and SynH+LT vs. SynH comparisons for different cell growth stages, with datasets restricted to upregulated (A) and downregulated (B) genes.
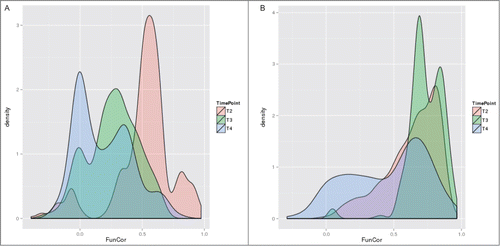
Figure 7. Distributions of correlation coefficients between vectors of -log10(GS-FDR) values computed for ACSH vs. SynH and SynH+LT vs. SynH comparisons for different count normalization methods, with data sets restricted to upregulated genes at exponential (T2) growth stage.
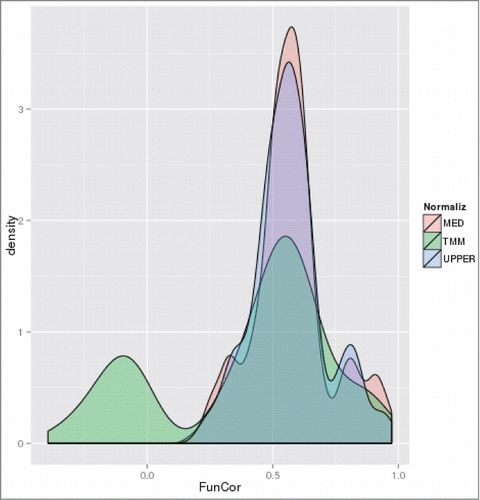