Figures & data
Figure 1. Single-cell perspectives frequently combine genomic, epigenomic, transcriptomic and spatial modalities to develop parallel “snapshots” of cellular function. Established antibody-omic techniques and target-agnostic proteomics are valuable complements that provide a holistic systems immunology perspective.
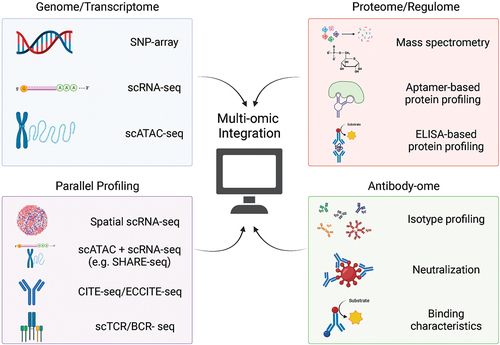
Figure 2. High-level conceptual schematics of common computational multi-omics datasets integration methods including integrated visualization (top panel), factor analysis and matrix factorization (middle panel), and neural network-based methods (bottom panel).
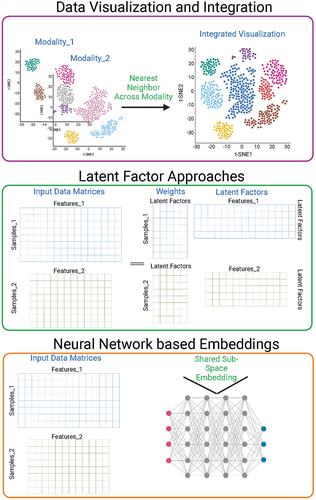
Figure 3. The top panel illustrates the application of methods like network propagation on biological networks guided by priors like expression and epigenetic information to uncover disease/trait relevant subnetworks or modules. The bottom panel depicts the recent use of generative deep learning models to integrate different biological networks in a transformed (reduced dimension) space.
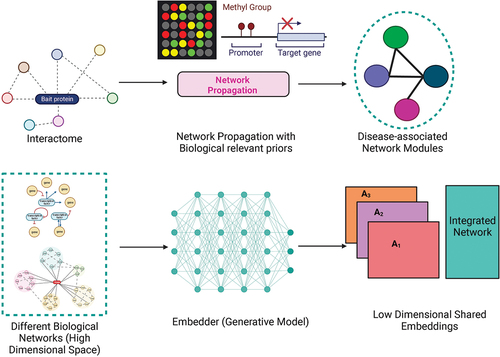