Figures & data
Figure 3. Functional enrichment analysis of the DEGs
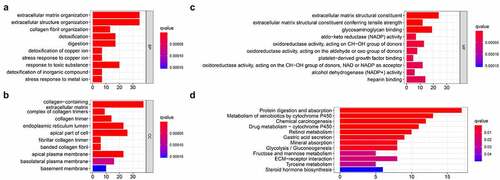
Figure 4. Prognosis-related gene screening
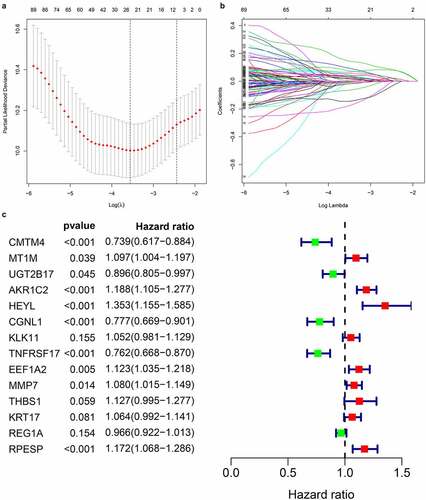
Figure 5. Prognostic analysis of 14-genes signature in the train cohort
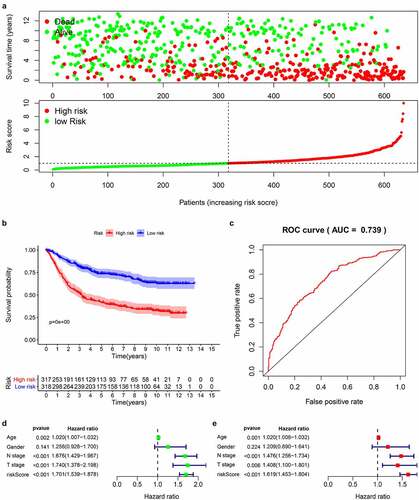
Figure 6. Prognostic analysis of 14 genes signature in the GSE15459 data set
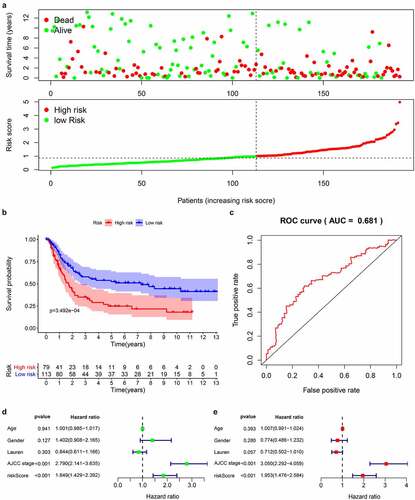
Figure 7. Risk and clinicopathological characteristics of 14 genes
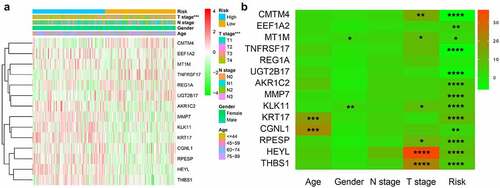
Figure 9. Establishment and validation of Nomogram (a) Nomogram for predicting 1–5 years OS of GC patients. (b) calibration chart for nomogram accuracy
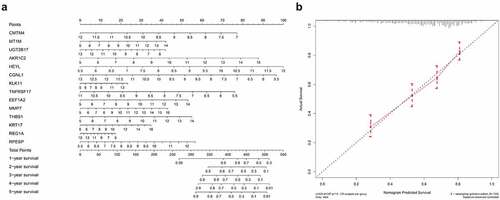