Figures & data
Figure 1. High-level schematic representation of the proposed framework. The top part refers to driver behaviour analysis; the lower part to driver behaviour simulation.
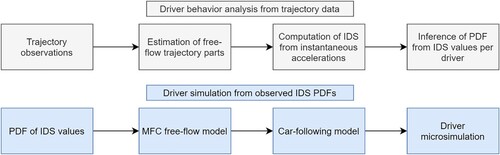
Figure 2. Identified sharp acceleration events corresponding to unconstrained vehicle movement in experimental observations.
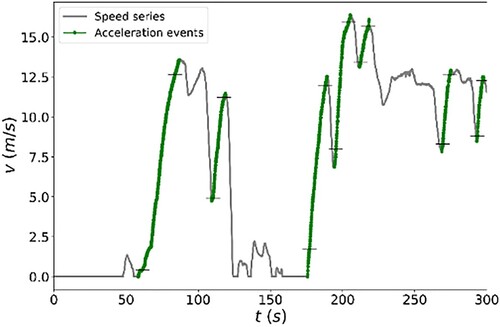
Figure 3. The unrealistic, feasible and common acceleration-speed domain [model representation from (He et al. Citation2020; Makridis et al. Citation2019)] for the vehicle used in the employed experimental campaign (see Section 2.4).
![Figure 3. The unrealistic, feasible and common acceleration-speed domain [model representation from (He et al. Citation2020; Makridis et al. Citation2019)] for the vehicle used in the employed experimental campaign (see Section 2.4).](/cms/asset/920c7b59-7aee-4495-91d8-0ae73d4b4350/ttrb_a_2125458_f0003_oc.jpg)
Figure 4. Naturalistic data refer to the 20 observed drivers of the experimental campaign used in this work. (a) shows acceleration over speed per movement and (b) the proposed values per speed per movement.
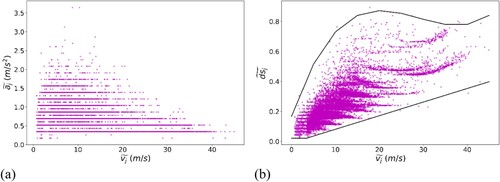
Figure 5. Uniform distribution of IDS values across the whole speed range. Data refer to 20 observed drivers ( denotes the ID of an acceleration event).
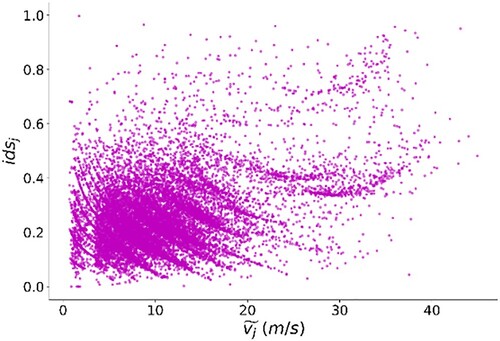
Table 1. Basic statistics of the trips per driver.
Figure 6. Histogram of the observed IDS values for each driver along with the fitted lognormal PDF. The vertical red lines denote the location of the median.
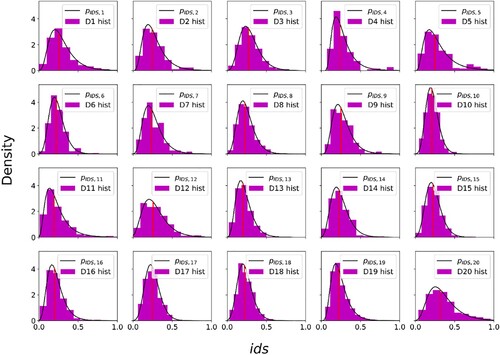
Figure 7. (a) K-means clustering result presented in a 3D plot, (b) PDFs of the inferred mild, normal and dynamic driver type.
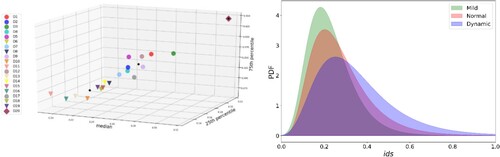
Figure 9. Reproduction of empirically-observed traffic oscillations with the MFC-LWR model as shown in (Makridis et al. Citation2020).
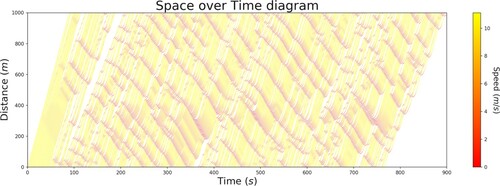
Figure 11. An indicative unconstrained movement per driver. For each event, the simulation area with the 25th and 75th percentile from the driver’s PDF is highlighted.
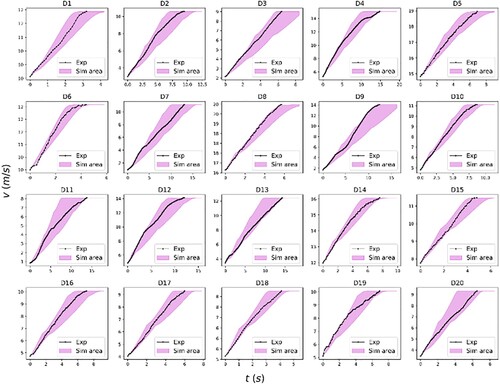
Table A1. Characteristics of fitted curves along with the special conditions for each one.
Table A2. The goodness of fit test results based on the K-S method.
Table A3. Parameters of the lognormal PDFs.