Figures & data
Figure 2. The whole routine of achieving elongated structure enhancement operation via left-invariant operation
based on the locally adaptive frame
. The exponential curve fit provides the LAD frame with full alignment to local structures. An orientation score
can be constructed from a 2D image
to an orientation score
via wavelet-type transform
, where we choose cake wavelets (Bekkers et al. 2014b)) for
.
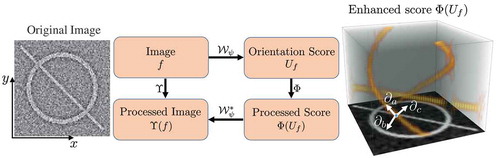
Figure 3. An example of the proposed optic disc (OD) and fovea detection. From Columns 1 to 5: original colour fundus image with manually annotated OD (black star) and fovea (white star), normalised green channel, Gaussian blurred image, PSEF response, and maximum response of PSEF indicating the OD location (red star) and the corresponding detected fovea (green star).

Table 1. The complete set of features extracted for each centreline pixel.
Table 2. Segmentation results on the DRIVE and STARE data sets.
Table 4. Comparisons of the success rate of the proposed framework for OD detection on all 1200 MESSIDOR images.
Table 5. Success rate of the proposed framework for fovea detection on the MESSIDOR data set compared with other methods.
Figure 4. Examples of A/V pixel-wise classifications by using different feature subsets. The last column shows the classifications using total 45 features including all the normalised intensities and the reflection features (Huang et al. (Citation2017a)). Blue indicates veins and red indicates arteries.
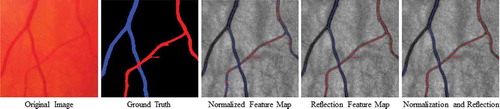
Figure 5. Two typical examples to show the vessel width-based artery and vein diameter ratio (AVR) difference in a specific ROI between one healthy subject and one diabetes type 2 subject. Blue indicates veins and red indicates arteries.
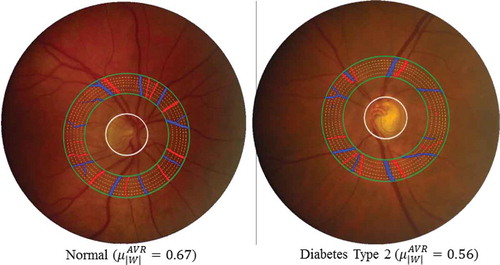
Figure 6. The stages of the proposed automatic width measurement technique: (a) centreline detection; (b) contour initialisation; (c) active contour segmentation; (d) obtaining the left and right edges; (e) Euclidean distance calculation; and (f) vessel calibre estimation.
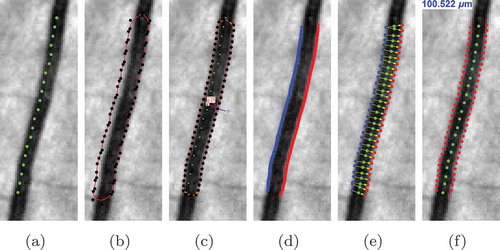
Figure 7. The vessels within the ROIs are used to compute the CRAE and CRVE values: (a) the widths of selected vessel are measured by the proposed method; (b) the six largest arteries and veins are then selected for the calculation of CRAE, CRVE and AVR values.
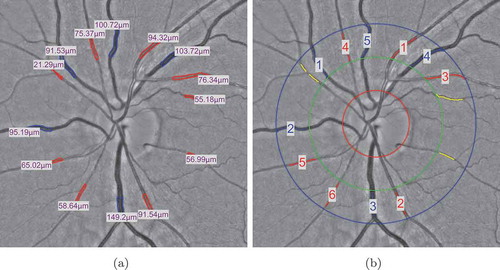
Figure 8. Validation of the exponential curvature estimation on a typical synthetic image. From left to right: input image (SNR = 1), ground truth colour-coded curvature map, measured curvature map with resp.
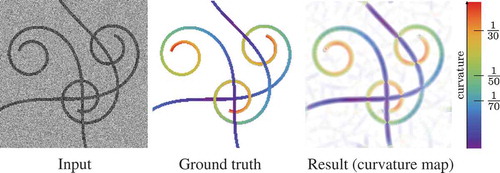
Figure 9. Examples to show the calculation of box dimension (BD) in a specific ROI between one healthy subject and one diabetes type 2 subject. Blue indicates veins and red indicates arteries.
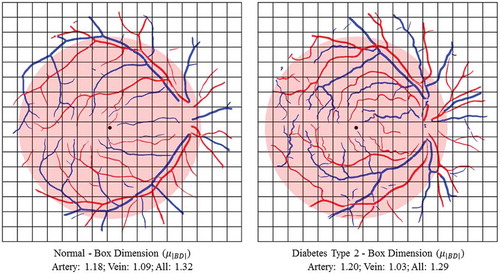
Figure 10. Examples of vessel segmentation by the proposed algorithm on images from the DRIVE and STARE data sets. Row 1: an image from the DRIVE data set (,
,
and
). Row 2: an image from the STARE data set (
,
,
and
). From Columns 1 to 4: original colour images, vessel enhancement result, hard segmentation result and ground truth.
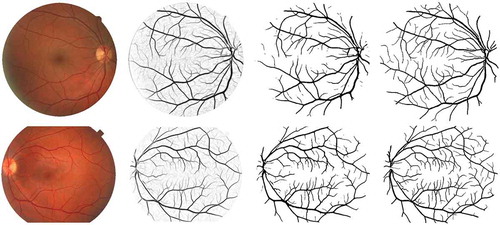
Table 3. The performance of the proposed framework on the DRIVE, INSPIRE, NIDEK, and Topcon data sets.
Figure 12. A/V classification results from the DRIVE and INSPIRE data sets. From left to right: the original images, the A/V label of the vessel centrelines, the pixel-wise classification and the segment-wise classification. Correctly classified arteries are in red, correctly classified veins are in blue and the wrongly classified vessels are in yellow.
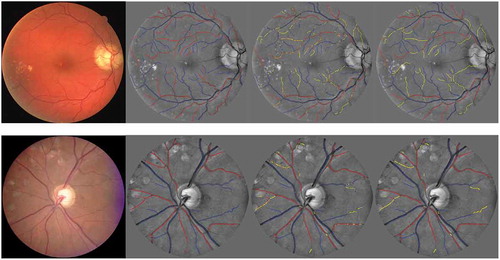
Figure 13. The Bland–Altman plots for comparing the (a) CRAE, (b) CRVE and (c) AVR values obtained by our method and the Vampire tool with the IVAN.
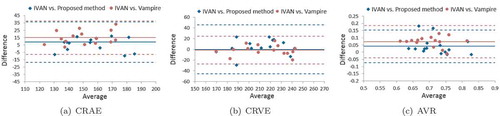
Figure 14. Two typical examples of showing the tortuosity (mean curvature) difference between one healthy subject and one diabetes type 2 subject. Blue indicates veins and red indicates arteries.
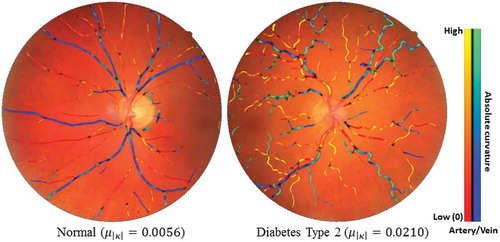
Table 8. The relative error of the CRAE, CRVE and AVR values obtained by the proposed method, IVAN and Vampire tools.
Table 6. Tortuosity measures and
in the MESSIDOR data set.
Table 7. The mean and standard deviation of FD values () for different DR grades.
Table 9. Comparison between FD values in different DR groups.
Table 10. The comparison of FD between two human observers and the automated vessel segmentation method.
Table 11. The comparison of values using different region of interest.