Figures & data
Figure 2. Each atlas is affinely registered to the target image
, and the obtained transformation is subsequently applied to the corresponding masks
. A majority voting filter (MV) produces an initial pre-segmentation mask
of cartilage-only labels and the Region of Interest (ROI) is finally defined as the morphological dilation of
.

Figure 3. Schematic representation of the feature description process for a regional SV , centered around a voxel
. Each SV comprises
patches, which in turn consist of
voxels. For all patches
, a feature vector
is calculated according to an encoding function (HOG, LBP, etc.), here denoted as
, where
serves as the individual descriptor for
. Finally, the whole
is characterized by
as the weighted aggregation of all its constituent descriptors.
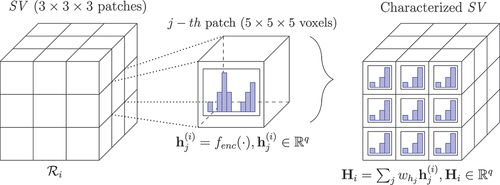
Table 1. Displacement vectors for GLCM and GLRLM for volumetric data.
Figure 4. Schematic representation of multi-view feature extraction for regional SV . Each one of the
patches is supplied with a feature vector for each descriptor(view) employed. The regional feature vector for each view is then calculated according to the process outlined in . The overall description of
is the collection of all
.
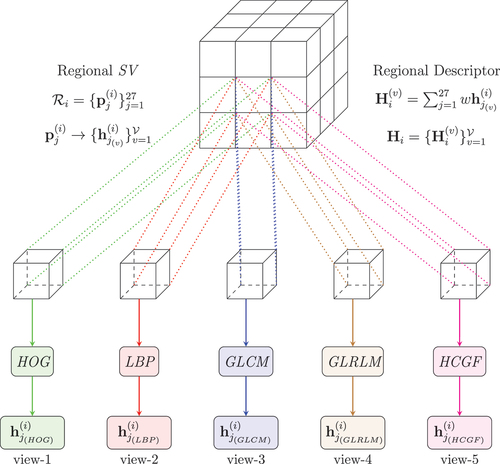
Figure 5. DESS, SPGR and T2 MRI sequences for the same subject. The differences in intensity profiles is apparent, especially between sequence T2 and the rest, suggesting that features learned from these images will yield sufficiently different descriptions of the image information content.
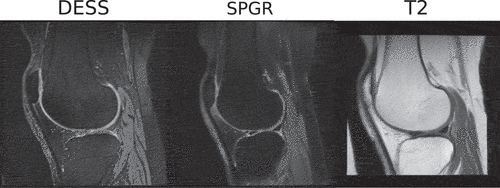
Figure 6. Out-of-sample label propagation: two sparse graphs are constructed for each view
of the out-of-sample batch
, connecting it to the global
and local
datasets respectively. The multi-view coefficients
are used to yield the final learned sparse graphs facilitating the labelling of voxels in
.
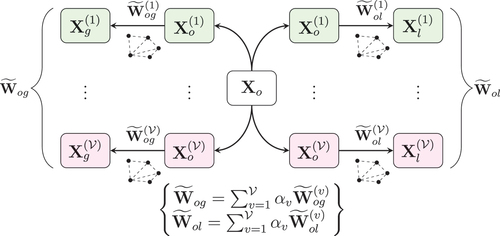
Table 2. Test cases examined for the first group of experiments.
Table 3. Performance comparison (mean and std. deviations) of all test cases for the proposed method of MV-HyLP. In case #1, GLM denotes the combination of GLCM, GLLM and All, the inclusion of all feature descriptors. Stacked and fused in case #2 refer to the list of feature descriptors (HOG, LBP, HCGF) being concatenated and fused through AMUSE, respectively. Finally, in case #3, Multi-feature corresponds exactly to the last row in case #1.
Figure 12. Segmentation results for femoral (FC) and tibial (TC) cartilage for the four instances of case #1 (left to right: Ground Truth, HOG, HOG & LBP, HOG & LBP & GLCM, all features). The first part of the figure illustrates a case of successful application of MV-KCS, while the second part presents in instance characterized by poor performance. In both cases, however, the positive effect of incorporating additional views is noticeable.
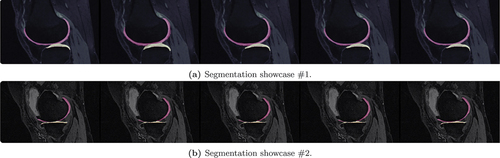
Table 4. Pairwise comparisons using the Nemenyi test for the cartilage results of the test cases in . Reported are the corresponding
-values. The binary indices in the subscript of each method’s name MV-HyLPxxxxx indicate the inclusion or exclusion of the respective feature descriptor HOG, LBP, GLCM, GLRLM, HCGF..
Table 5. Summary of segmentation performance measures (means and std. deviations) on the two cartilage classes of our proposed method MV-RegLP and its modification MV-HyLP, compared to Patch-Based Sparse-Coding (PBSC), Patch-Based Sparse-Coding with stacked features (PBSC(stacked)), Patch-Based joint label fusion (PB-JLF), Triplanar CNN, SegNet, MM-CNN-FC, VoxResNet, DenseVoxNet, KCB-Net, CAN3D, 3D-CNN + SSM, DAN, UA-MT and SS-DTC. The hyperparameter values for MV-RegLP and MV-HyLP are those obtained after parameter sensitivity analysis in previous section 8.3.
Table 6. Pairwise comparisons using the Nemenyi test for the cartilage results of the competing methodologies of . Reported are the corresponding
-values.
Data availability statement
Due to the nature of the research, due to [ethical/legal/commercial] supporting data is not available.