Figures & data
Table 1. Identification/classification of cataract and glaucoma using deep learning methods.
Figure 8. Ocular disease classification using SqueezeNet (batch size-6, optimizer-Adam) (with augmented image data).
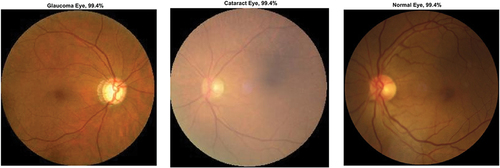
Figure 9. Ocular disease classification using darknet-53 (batch size-6, optimizer-sgdm) (with augmented image data).
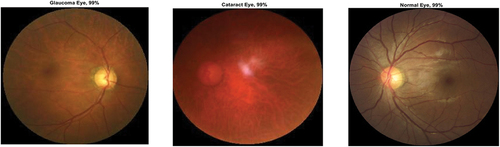
Figure 10. Ocular disease classification using EfficientNet-b0 (batch size-6, optimizer-sgdm) (with augmented image data).
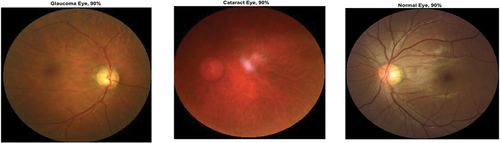
Figure 11. Effect of batch size and optimizer type on the ocular disease classification accuracy for various deep learning-based CNN (with data augmentation).
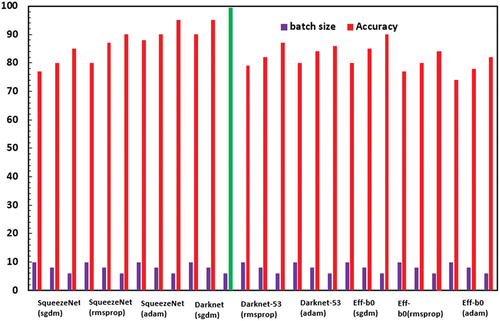
Table 2. Accuracy of various optimised networks.
Figure 14. 99.4% accuracy of darknet-53 (batch size-6, optimizer-SGDM) obtained during the training process.
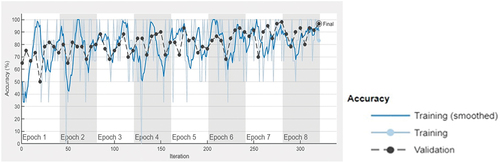
Figure 15. Loss function of the optimized darknet-53 model (batch size-6, optimizer-SGDM) obtained during the training process.
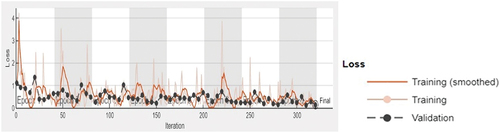
Table 3. Performance metrics of the optimised darknet-53 (batch size-6, optimiser-SGDM).