Figures & data
Figure 1. Map of New South Wales with the sample UCLs.
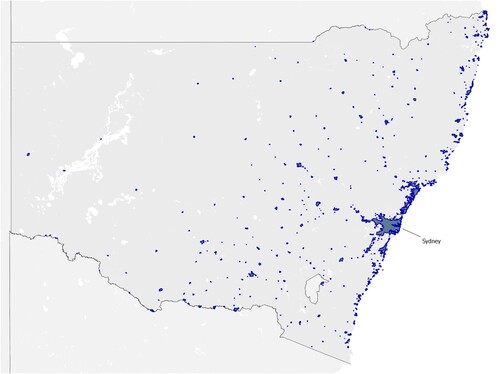
Figure 2. Maps showing SA1-level population and employment density in 2016 in the Sydney UCL.
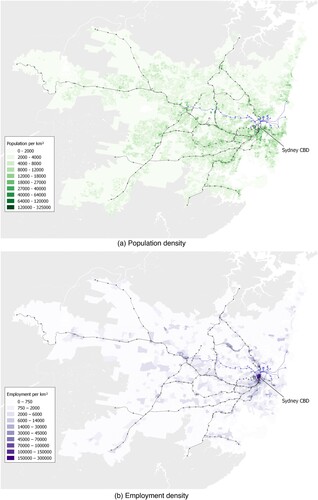
Figure 3. Maps showing SA1-level median residential property prices in the Sydney UCL in 2019 and 2021, both in 2019 dollars.
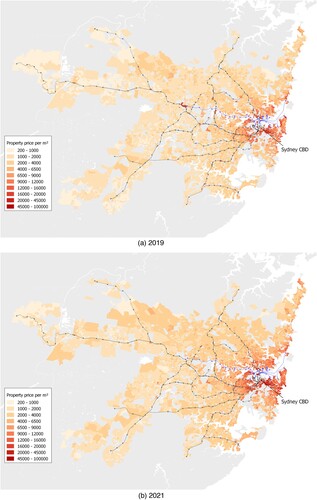
Table 1. Summary statistics for the main variables in the UCL-level dataset.
Table 2. Summary statistics for the main variables in the Sydney SA1-level dataset.
Figure 4. Quarterly median property prices per square metre from 2016 to June 2023, normalised to the first quarter of 2020. The plots compare towns or cities in New South Wales by population and neighbourhoods in the Sydney metropolitan area by road travel time to the CBD.
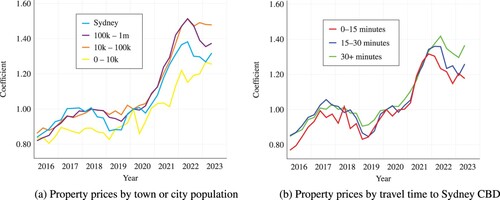
Table 3. OLS estimation results for the UCL-level relationships between (log) residential property prices by year and the (log) UCL population, distance to Sydney, and distance to the coast, with the populations and distances in separate regressions.
Figure 5. Coefficients on (log) UCL population and distances to Sydney and the coast, from separate regressions.
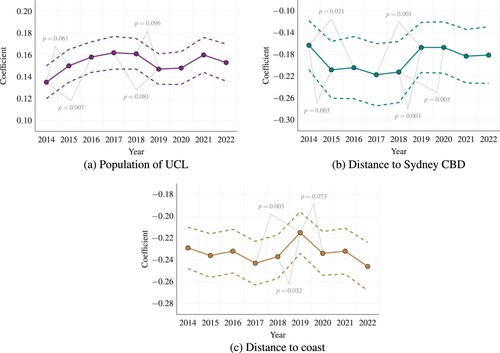
Figure 6. Coefficients on (log) UCL population and distances to Sydney and the coast, from the same regression for each year.
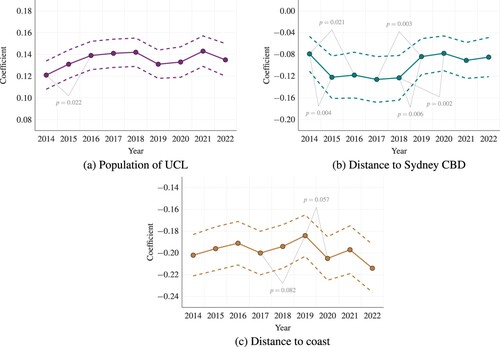
Table 4. OLS estimation results for the UCL-level relationships between (log) residential property prices by year and the (log) UCL population, distance to Sydney, and distance to the coast, with the populations and distances in the same regression for each year.
Table 5. OLS estimation results for the UCL-level relationships between (log) residential property prices in 2019 and 2021 and the first two moments of (log) UCL population, distance to Sydney and distance to the coast.
Figure 7. Coefficients on (log) travel times by road to the Sydney CBD, the beach and Sydney Harbour and by rail or ferry to the CBD, from separate regressions.
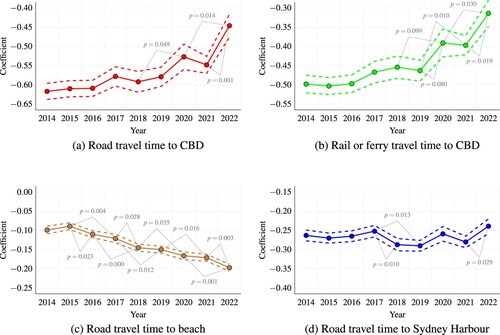
Table 6. OLS estimation results for the SA1-level relationships between (log) residential property prices by year in the Sydney metropolitan area and each type of (log) travel time, with the travel times in separate regressions.
Figure 8. Coefficients on (log) travel times by road to the Sydney CBD, the beach and Sydney Harbour and by rail or ferry to the CBD, from the same regression for each year.
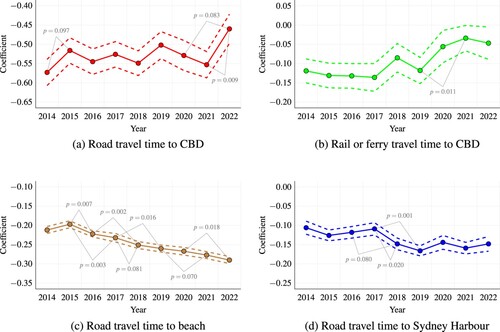
Table 7. OLS estimation results for the SA1-level relationships between (log) residential property prices by year in the Sydney metropolitan area and each type of (log) travel time, with all four types of travel time in the same regression for each year.
Supplemental Material
Download PDF (850.5 KB)DATA AVAILABILITY STATEMENT
The data used in this research were assembled from several public sources, as detailed in the Data section above, and are free to download with no permission required. The primary sources were the New South Wales Valuer General and the Australian Bureau of Statistics. Other data were from the Open Source Routing Machine, Transport for NSW, Geoscience Australia, the Bureau of Meteorology, and the New South Wales Bureau of Crime Statistics and Research.