Figures & data
Table 1. Demand parameters by layout and product type
Table 2. Process parameters of the first layout
Table 3. Model 2 – summary of time requirement parameters for data generation
Table 4. Process parameters of the second layout
Table 5. Model 2 – summary of time requirement parameters for data generation
Table 6. Parameters of the SKUs in Model 3
Table 7. Model 3 – matrix of data features
Figure 7. Model 3 – total product assembled by SKU in each cell per day and assembly cell utilisation.
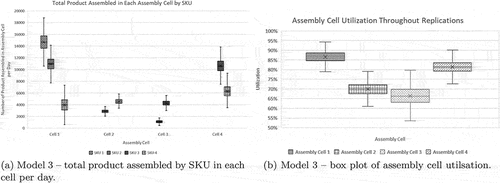
Table 8. Model 3 – summary of time requirement parameters for data generation