Figures & data
Figure 1. The globally spatial and seasonal distributions of SFTS during 2010–2018. (A) The average annual incidence and the number of SFTS cases were indicated at the provincial level. (B) The seasonality is presented as a radar diagram for each of three mainly affected countries by SFTS, including China, Japan and South Korea. The circumference is divided into 12 months in a clockwise direction, and the radius represents average monthly incidences over 2010–2018.
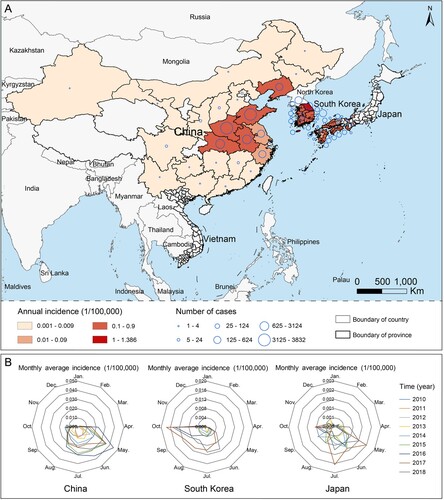
Table 1. Baseline demographic characteristics of reported SFTS patients in China, Japan and South Korea from 2010 to 2018.
Figure 2. Global recorded locations and model-predicted distribution probability of H. longicornis. (A) Each occurrence record of H. longicornis was geo-referenced and linked to the digital world map. The recording time of the H. longicornis presence was marked by colour gradients from red to green. (B) The potential geographic range of H. longicornis was predicted and mapped by using a maximum entropy method based on eco-geographical and climatic variables.
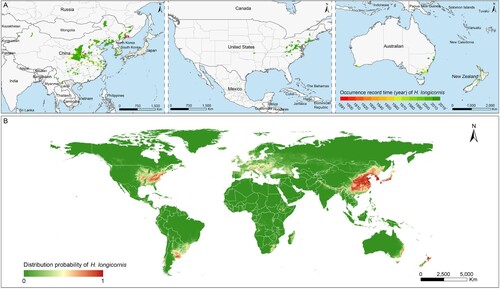
Table 2. The contribution of environmental variables to predict the global risk probability of H. longicornis presence based on ecological niche model.
Figure 3. ROC curves for the risk probability of SFTS transmission based on climatic, eco-geographical and social variables by using BRT models. (A) The red curves are average predicted lines for risk factors by 100 repeats (grey lines) based on the bootstrapping procedure. (B) The grey lines are the ROC curve for 100 repeats, and the red, blue and black lines indicate the average ROC curves of 100 repeats based on the bootstrapping procedure for the train set, test set and prediction, respectively.
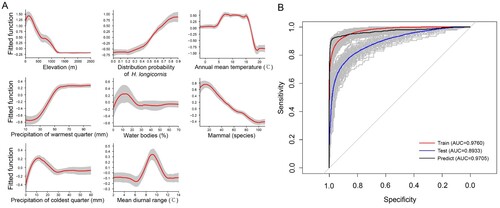