Figures & data
Figure 1. Apple leaf diseases considered in this study: (a) powdery mildew, (b) Alternaria, and (c) rust disease.
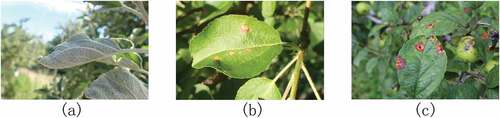
Figure 2. Framework of the FPN–ISResNet–Faster RCNN model, where C2–C5 represent the predicted feature layers, in represents the inception module, P2–P5 represent the predicted outputs of the feature graphs from the FPN, ROI represents the region of interest, and Cls_score represents the classification output by softmax.
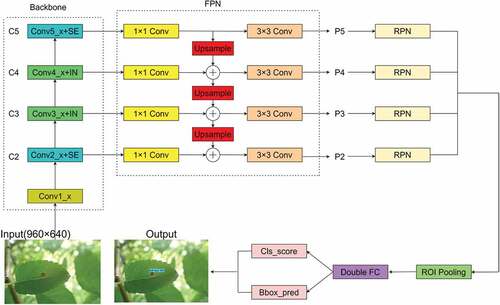
Table 1. ISResNet structure.
Table 2. Different reduction coefficients (r) obtained from SE-Resnet-50 to determine its best value (J. Hu et al., Citation2020).
Table 3. Comparison of the accuracies and speeds of different models for detecting apple leaf diseases.
Figure 3. Visualized detection results of (A) powdery mildew, (B) Alternaria leaf spots, (C) rust disease, and (D) Alternaria leaf spots and rust disease in apple tree leaves using the FPN–ISResNet–Faster RCNN model. (E) Identification errors in the left image labeled with (1) and omissions in the right image labelled with (2).
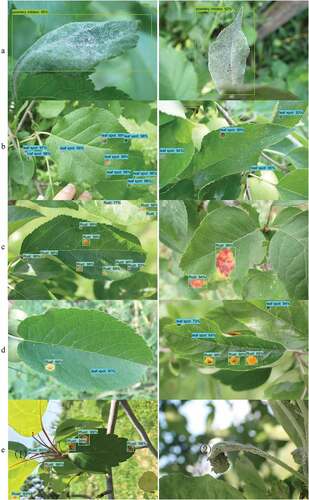
Figure 4. A UAV image of the apple orchard (e) with four sampled apple trees (a, b, c, and, d) in Shatan village, Enhe Town, Zhongning County, Ningxia, China acquired at 16:00 on July 8, 2021.
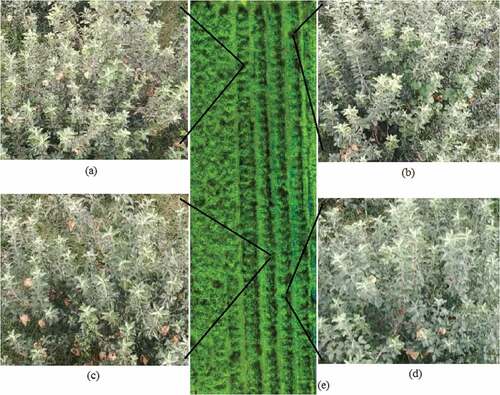
Figure 5. Distribution of powdery mildew, Alternaria leaf spots, and rust disease in apple tree leaves in magnified images of the (a) northern and (b) southern orchard.
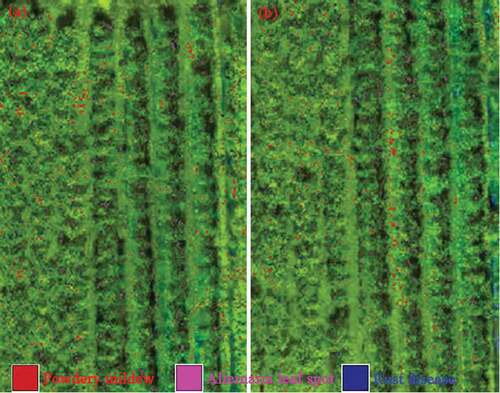
Table 4. Precision rates of feature extraction networks used to detect apple leaf diseases.
Table 5. Accuracies of ResNet50 with different positions and numbers of SENet modules after the convolution layer for the detection of apple leaf diseases.
Table 6. Accuracies of ResNet50 with different positions and numbers of inception modules after the convolution layers for the detection of apple leaf diseases.
Table 7. Comparison of detection accuracies of apple leaf diseases obtained from models with and without FPNs.
Table 8. Comparison of detection accuracies of apple leaf diseases obtained by the proposed model with different scales of anchor frames.
Data availability statement
Dataset(s) derived from public resources and made available with the article. https://www.kaggle.com/competitions/plant-pathology-2021-fgvc8/data