Figures & data
Figure 1. Classic pipeline for decoding visual information. First the neural signal is measured whilst the individual performs a visual imagery or perception task. This neural signal is then decoded. The output contents include an example of pictorial reconstruction (a), semantic classification (b), and identification of low level visual information such as shape (c) and colour (d).
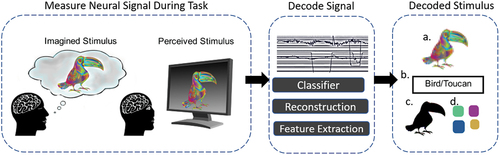
Figure 2. This demonstrates the relative speed taken to process similar information content in either a. visual or b. auditory modalities. Object recognition, such as this glacier scene, can be detected as early as 100ms from visual perception, figure taken and adapted from Lowe et al. [Citation29]. How long does it take to say the sentence out loud in B?
![Figure 2. This demonstrates the relative speed taken to process similar information content in either a. visual or b. auditory modalities. Object recognition, such as this glacier scene, can be detected as early as 100ms from visual perception, figure taken and adapted from Lowe et al. [Citation29]. How long does it take to say the sentence out loud in B?](/cms/asset/6e5cdaad-75c2-4e60-a1ad-f3fbb1fecfbc/tbci_a_2287719_f0002_oc.jpg)
Figure 3. This demonstrates key regions related to visual processing, such as V1–3 and the lateral geniculate nucleus.This image is adapted and taken from BodyParts3D, © the Database center for Life Science licensed under CC attribution-share alike 2.1 Japan.
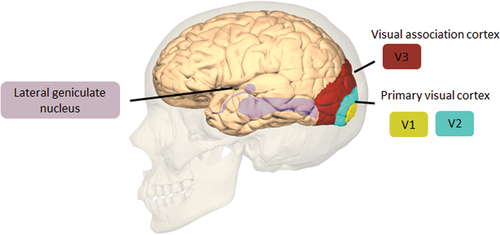
Table 1. Key words used to identify papers included in the following literature review for each target.
Table 4. Simple visual features EEG datasets.
Figure 4. Types of stimuli used in the three mentioned colour decoding studies; a) shows examples of coloured Gabor patch stimuli, b) depicts the coloured square on a curved projector in Rasheed [Citation51] and c) shows the Arduino with led lights used in Yu and Sim [Citation49]. B and C images are adapted from their respective papers.
![Figure 4. Types of stimuli used in the three mentioned colour decoding studies; a) shows examples of coloured Gabor patch stimuli, b) depicts the coloured square on a curved projector in Rasheed [Citation51] and c) shows the Arduino with led lights used in Yu and Sim [Citation49]. B and C images are adapted from their respective papers.](/cms/asset/fb42c872-18f4-4c32-9c42-cab4ccc57f1b/tbci_a_2287719_f0004_oc.jpg)
Table 2. Decoding performance metrics for colour, including details on the classifier employed, feature extraction methods, spatial areas selected, chosen time windows, and the calculation of information transfer rate (ITR).
Figure 5. In this figure depicted are: a. The geometric shapes used in [Citation46] b. seven geometric shapes from [Citation44].
![Figure 5. In this figure depicted are: a. The geometric shapes used in [Citation46] b. seven geometric shapes from [Citation44].](/cms/asset/cfd85c14-aa7a-4d71-ba7c-2de7585563ad/tbci_a_2287719_f0005_b.gif)
Table 3. Decoding performance metrics for spatial features, including details on the classifier employed, feature extraction methods, spatial areas selected, chosen time windows, and the calculation of information transfer rate (ITR).
Figure 6. a) shows reconstructions using MVAE wakita et al. [Citation70] and b) are reconstructions from Orima and Motoyoshi [Citation71] using the figure is adapted from Orima and Motoyoshi [Citation71]; wakita et al. [Citation70].
![Figure 6. a) shows reconstructions using MVAE wakita et al. [Citation70] and b) are reconstructions from Orima and Motoyoshi [Citation71] using the figure is adapted from Orima and Motoyoshi [Citation71]; wakita et al. [Citation70].](/cms/asset/f1479ad7-307e-493f-9a85-cfb0e330f311/tbci_a_2287719_f0006_oc.jpg)
Table 5. Decoding performance metrics for complex stimuli, including details on the classifier employed, feature extraction methods, spatial areas selected, chosen time windows, and the calculation of information transfer rate (ITR).
Table 6. Datasets for naturalistic/complex stimuli relaying stimuli details, acquisition device, sampling rate and duration of the imagination or perception task.
Figure 7. Examples of the unfamiliar male faces used. The top row are the original displayed faces, the bottom row are their respective reconstructions. Adapted from Nemrodov et al. [Citation73].
![Figure 7. Examples of the unfamiliar male faces used. The top row are the original displayed faces, the bottom row are their respective reconstructions. Adapted from Nemrodov et al. [Citation73].](/cms/asset/8a5cec1e-34f9-4057-a7d8-0a5c38dc50c3/tbci_a_2287719_f0007_oc.jpg)
Figure 8. Showing the types of binary stimuli used. In a) classification of an imagined/perceived hammer vs. A flower adapted from Kosmyna et al. [Citation75], b) classification of Sydney Harbour bridge vs. Santa Claus adapted from Shatek et al. [Citation74].
![Figure 8. Showing the types of binary stimuli used. In a) classification of an imagined/perceived hammer vs. A flower adapted from Kosmyna et al. [Citation75], b) classification of Sydney Harbour bridge vs. Santa Claus adapted from Shatek et al. [Citation74].](/cms/asset/06335c25-4558-40f8-9d9b-25b83374ce04/tbci_a_2287719_f0008_oc.jpg)
Figure 9. Examples of reconstruction results from Zheng et al. [Citation84].
![Figure 9. Examples of reconstruction results from Zheng et al. [Citation84].](/cms/asset/d7832779-410a-4b64-8df6-05506e121d42/tbci_a_2287719_f0009_oc.jpg)
Figure 10. Illustrates that at the time of this review, at least eight further visual decoding studies have been carried out which use Spampinato et al. [Citation76]’s foundational dataset.
![Figure 10. Illustrates that at the time of this review, at least eight further visual decoding studies have been carried out which use Spampinato et al. [Citation76]’s foundational dataset.](/cms/asset/733eb520-6655-46f4-9b28-b9e1848c1876/tbci_a_2287719_f0010_b.gif)
Table 7. This table compares the relative cost, spatial and temporal resolution, portability, and invasiveness of the neuroimaging modalities discussed in this current section on EEG hardware.
Figure 11. The left side depicts the difference between unstructured, structured and dynamic visual noise. For unstructured dynamic, black and white dots are selected at random with multiple iterations. This example shows three random dot variations shown consecutively. The right hand side depicts how visual noise can be injected into the experiment pipeline, before and after each perception and imagery task.
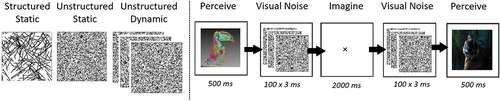