Figures & data
Table 1. The limitation of used parameters in shrinkage and creep prediction models
Table 2. The parameters and its variation range which is used for configuration of networks
Table 3. Evaluation of the different training algorithm
Figure 2. The linear regression curves of primary networks configured with different training algorithms
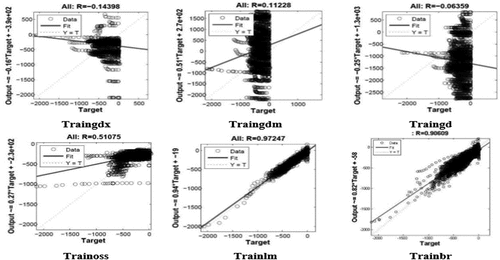
Table 4. Specifications of configured networks with different neurons numbers with Levenberg–Marquardt and Bayesian framework algorithms
Table 5. The specimens mix proportions
Table 6. Distribution of the different shrinkage models error
Table 7. Distribution of the shrinkage prediction error
Figure 7. Comparison of shrinkage strain predicted by neural networks technique with experimental observations for A300 and B300 mixtures
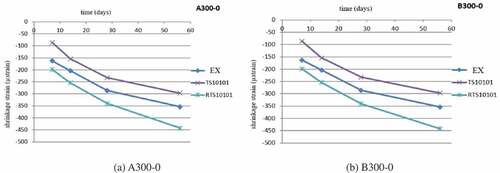
Figure 8. Comparison of shrinkage strain predicted by neural networks technique with experimental observations for A300-15 and B300-15 mixtures
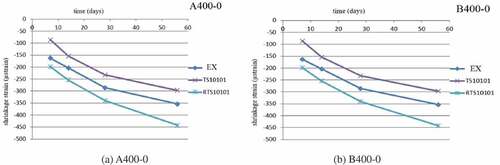