Figures & data
Table 1. Analysis of data sample
Figure 2. Combinatory influence of the utilized genetic algorithm (a) penalty factor at various iteration (b) Epsilon as a function of iteration (c) kernel mapping parameter as number of iteration progresses (d) error convergence at different iteration.
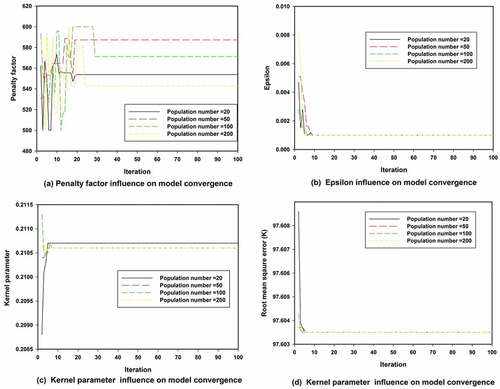
Table 2. Results of the genetic algorithm (SVR parameters)
Table 3. Extreme learning machine weights for model reproducibility
Figure 4. Predictive capacity of developed models (a) using RMSE for training dataset (b) using MAE for training dataset (c) using RMSE for testing dataset (d) using MAE for testing dataset.
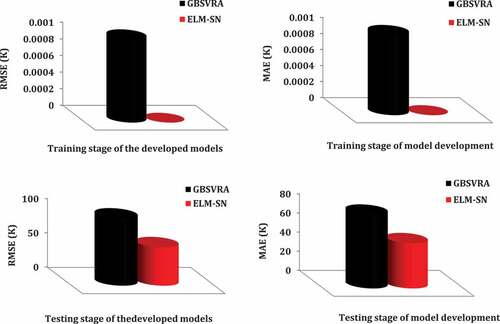
Table 4. Performances of the developed intelligent models
Figure 5. Predictions of the developed intelligent models for different spinel ferrite magnetocaloric compounds [S1 = Zn0.6Cu0.4Fe2O4 (F. Hcini et al., Citation2021), S2 = Zn0.4Ni0.2Cu0.4Fe2O4 (F. Hcini et al., Citation2021), S3 = Zn0.250Ni0.250Mg0.5Fe2O4 (F. Hcini et al., Citation2021), S4 = Ni0.7Zn0.3Fe2O4 (Bahhar et al., Citation2021), S5 = Ni0.5Zn0.5Fe2O4 (Oumezzine et al., Citation2015), S6 = Ni0.3Zn0.7Fe2O4 (Oumezzine et al., Citation2015), and S7 = Cu0.4Zn0.6Fe2O4 (Bahhar et al., Citation2021)].
![Figure 5. Predictions of the developed intelligent models for different spinel ferrite magnetocaloric compounds [S1 = Zn0.6Cu0.4Fe2O4 (F. Hcini et al., Citation2021), S2 = Zn0.4Ni0.2Cu0.4Fe2O4 (F. Hcini et al., Citation2021), S3 = Zn0.250Ni0.250Mg0.5Fe2O4 (F. Hcini et al., Citation2021), S4 = Ni0.7Zn0.3Fe2O4 (Bahhar et al., Citation2021), S5 = Ni0.5Zn0.5Fe2O4 (Oumezzine et al., Citation2015), S6 = Ni0.3Zn0.7Fe2O4 (Oumezzine et al., Citation2015), and S7 = Cu0.4Zn0.6Fe2O4 (Bahhar et al., Citation2021)].](/cms/asset/a07ee3d5-b81b-4276-b96e-a49052309e5b/oaen_a_2172790_f0005_oc.jpg)
Figure 6. Comparison of the estimates of the developed intelligent models for classes of spine ferrite magnetocaloric compounds [S8 = Cu0.2Zn0.8Fe2O4 (Oumezzine et al., Citation2015), S9 = Ni0.4Zn0.6Fe2O4 (Oumezzine et al., Citation2015), S10 = Ni0.35Zn0.65Fe2O4 (Oumezzine et al., Citation2015), S11 = Ni0.3Zn0.7Fe2O4 (Oumezzine et al., Citation2015), S12 = Ni0.25Zn0.75Fe2O4 (Oumezzine et al., Citation2015), S13 = MnCeFeO4 (Bahhar et al., Citation2021), S14 = CoCeFeO4 (Bahhar et al., Citation2021)].
![Figure 6. Comparison of the estimates of the developed intelligent models for classes of spine ferrite magnetocaloric compounds [S8 = Cu0.2Zn0.8Fe2O4 (Oumezzine et al., Citation2015), S9 = Ni0.4Zn0.6Fe2O4 (Oumezzine et al., Citation2015), S10 = Ni0.35Zn0.65Fe2O4 (Oumezzine et al., Citation2015), S11 = Ni0.3Zn0.7Fe2O4 (Oumezzine et al., Citation2015), S12 = Ni0.25Zn0.75Fe2O4 (Oumezzine et al., Citation2015), S13 = MnCeFeO4 (Bahhar et al., Citation2021), S14 = CoCeFeO4 (Bahhar et al., Citation2021)].](/cms/asset/bc4f5659-f177-40d4-849d-2d2561881bcf/oaen_a_2172790_f0006_oc.jpg)
Figure 7. Magnetic ordering temperature of spinel ferrite magnetocaloric compounds obtained from developed intelligent models [S15 = Ni0.4Mg0.3Cu0.3Fe2O4, S16 = Cu0.3Zn0.7Fe2O4 (Bahhar et al., Citation2021), S17 = Mg0.5Zn0.5Fe2O4 (Fortas et al., Citation2020), S18 = Mg0.3Zn0.7Fe2O4 (Bouhbou et al., Citation2022),S19 = Mg0.2Zn0.8Fe2O4 (Bouhbou et al., Citation2022), S20 = Zn0.7Ni0.1Cu0.2Fe2O4 (Bouhbou et al., Citation2022), S21 = Mg0.35Zn0.65Fe2O4 (Bouhbou et al., Citation2022)].
![Figure 7. Magnetic ordering temperature of spinel ferrite magnetocaloric compounds obtained from developed intelligent models [S15 = Ni0.4Mg0.3Cu0.3Fe2O4, S16 = Cu0.3Zn0.7Fe2O4 (Bahhar et al., Citation2021), S17 = Mg0.5Zn0.5Fe2O4 (Fortas et al., Citation2020), S18 = Mg0.3Zn0.7Fe2O4 (Bouhbou et al., Citation2022),S19 = Mg0.2Zn0.8Fe2O4 (Bouhbou et al., Citation2022), S20 = Zn0.7Ni0.1Cu0.2Fe2O4 (Bouhbou et al., Citation2022), S21 = Mg0.35Zn0.65Fe2O4 (Bouhbou et al., Citation2022)].](/cms/asset/3e0aa486-f4cc-41e0-93b2-2497afb53552/oaen_a_2172790_f0007_oc.jpg)
Figure 8. Estimated MOT for different spinel ferrite magnetocaloric compounds [S22 = Zn0.375Ni0.125Mg0.5Fe2O4 (Fortas et al., Citation2020), S23 = Zn0.250Ni0.250Mg0.5Fe2O4 (Fortas et al., Citation2020), S24 = Mg0.6Cu0.2Ni0.2Fe2O4 (Fortas et al., Citation2020),S25 = Mg0.6Cu0.4Fe2O4 (Fortas et al., Citation2020), S26 = Ni0.4Cd0.3Zn0.3Fe2O4 (Fortas et al., Citation2020),S27 = Ni0.6Cd0.2Zn0.2Fe2O4 (Fortas et al., Citation2020), S28 = Zn0.7Ni0.3Fe2O4 (Oumezzine et al., Citation2015), S29 = Zn0.7Ni0.2Cu0.1Fe2O4 (Fortas et al., Citation2020)].
![Figure 8. Estimated MOT for different spinel ferrite magnetocaloric compounds [S22 = Zn0.375Ni0.125Mg0.5Fe2O4 (Fortas et al., Citation2020), S23 = Zn0.250Ni0.250Mg0.5Fe2O4 (Fortas et al., Citation2020), S24 = Mg0.6Cu0.2Ni0.2Fe2O4 (Fortas et al., Citation2020),S25 = Mg0.6Cu0.4Fe2O4 (Fortas et al., Citation2020), S26 = Ni0.4Cd0.3Zn0.3Fe2O4 (Fortas et al., Citation2020),S27 = Ni0.6Cd0.2Zn0.2Fe2O4 (Fortas et al., Citation2020), S28 = Zn0.7Ni0.3Fe2O4 (Oumezzine et al., Citation2015), S29 = Zn0.7Ni0.2Cu0.1Fe2O4 (Fortas et al., Citation2020)].](/cms/asset/d826dfd4-7f74-4df0-b1dd-a23431e82b12/oaen_a_2172790_f0008_oc.jpg)
Data availability statement
section 3.2 contained the details of the raw data utilized for these findings with their references.