Figures & data
Table 1. Comparison of existing ANN models used in RC beam analysis
Table 2. Comparison of existing ANN models used in RC beam analysis (Continued)
Table 3. The input datasets taken from various literatures (Belletti et al., Citation2020; Dai et al., Citation2020; Dai, Chen, Wang, Ma et al., Citation2021, Citation2021; Dai et al., Citation2016; ElBatanouny et al., Citation2015; Jeon et al., Citation2020; Liu & Fan, Citation2019; Lu et al., Citation2021; Menoufy & Soudki, Citation2014; Moawad, El-Karmoty et al., Citation2018; Moawad, Mahmoud et al., Citation2018; Papãľ & Melchers, Citation2011; Rinaldi et al., Citation2010; Saraswathy et al., Citation2017; Zeng et al., Citation2010; Zhang, Wang, Zhang, Liu et al., Citation2017)
Table 4. The statistical measurements of input datasets
Table 5. The statistical measurements of input datasets (Continued …)
Figure 4. The proposed RBPBTNN models for ultimate load, ultimate moment and deflection predictions.
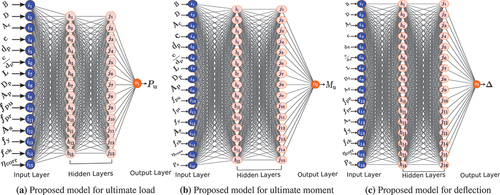
Figure 5. The proposed RBPBTNN training performance comparison when K-fold and training repetitions are varied.
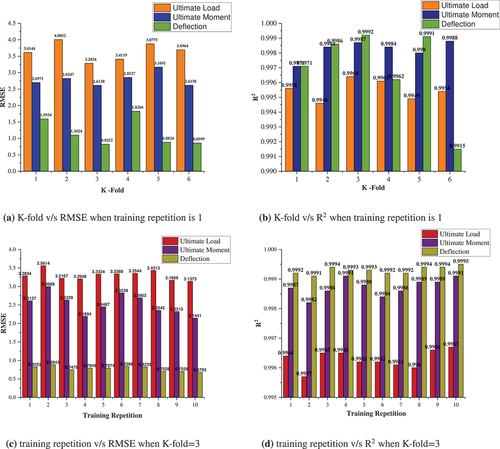
Table 6. Parametric details of the proposed ANN models during training and validation phases
Table 7. Performance comparison of the proposed RBPBTNN with other ANN models on testing data
Figure 6. Parametric analysis of input parameters on ultimate load, ultimate moment and deflection predictions.
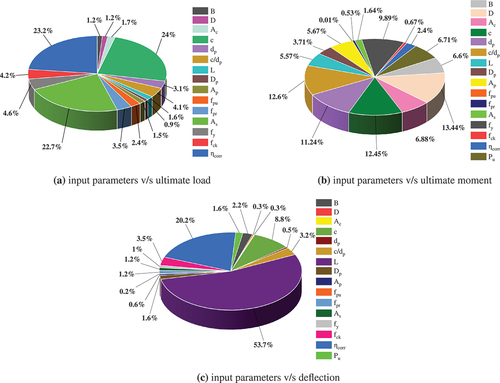