Figures & data
Table 1. Limits of each feature used to prepare the concrete.
Table 2. Statistical characteristics of experimental data.
Figure 6. Compressive strength of observed and predicted against testing sample of (a) random forest regression and (b) AdaBoost regression.
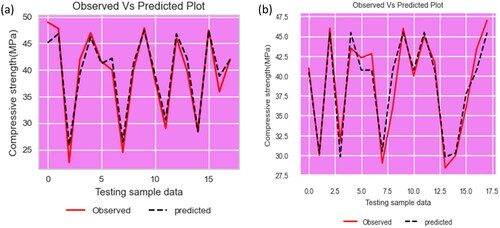
Table 3. Training and testing measures of regression models.
Figure 7. Comparison of performance metrics of (a) training and (b) testing for random forest regression and AdaBoost regression.
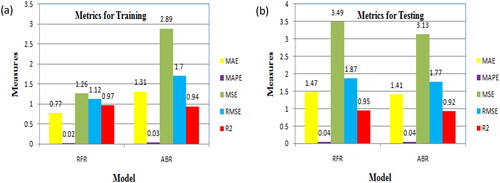
Figure 8. Comparison of prediction error plots for (a) random forest regression and (b) AdaBoost regression.
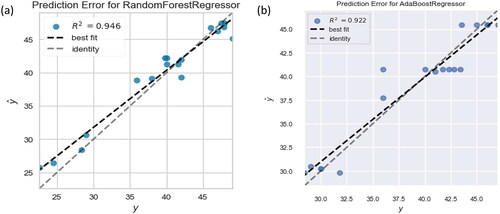
Figure 9. Comparison of residual plots for (a) random forest regression and (b) AdaBoost regression.
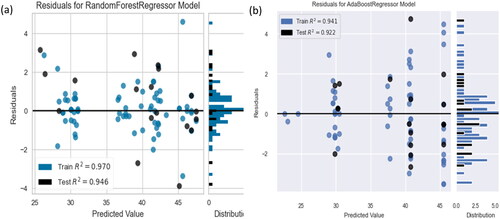
Data availability statement
The data that support the findings of this study are available from the corresponding author, [C Vivek Kumar], upon reasonable request.