Figures & data
Figure 1. Survival function of the zero-inflated cure rate model as presented in Louzada, Oliveira, and Moreira (Citation2015).
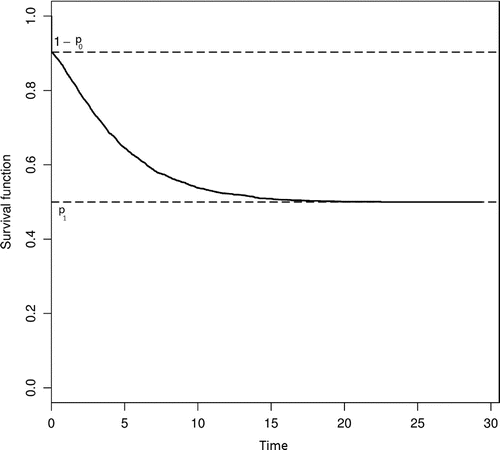
Figure 2. Bias, square root of mean squared error and coverage probability (CP) of the maximum likelihood estimation ,
,
,
of zero-inflated promotion cure rate regression model for simulated data under the three scenarios of parameters, obtained from Monte Carlo simulations with 1,000 replications and increasing sample size (n). 1 indicates scenario 1 with characteristic of having a low rate of STD and non-default. 2 indicates the scenario 2 with characteristic of having a moderate rate of STD and non-default. 3 indicates scenario 3 with a characteristic of having a high rate of STD and non-default.
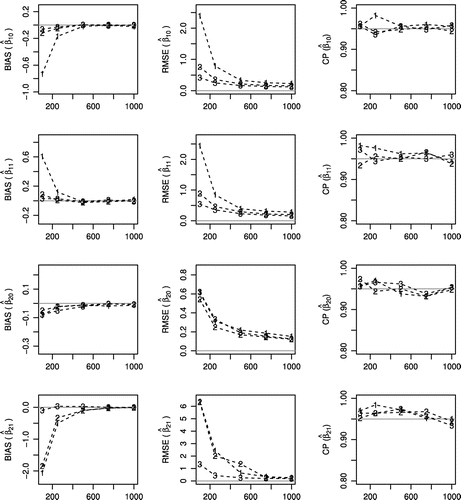
Figure 3. Bias, square root of mean squared error and coverage probability (CP) of the maximum likelihood estimation ,
,
,
of zero-inflated promotion cure rate regression model for simulated data under the three scenarios of parameters, obtained from Monte Carlo simulations with 1,000 replications and increasing sample size (n). 1 indicates the scenario 1 with characteristic of having a low rate of STD and non-default. 2 indicates the scenario 2 with characteristic of having a moderate rate of STD and non-default. 3 indicates scenario 3 with characteristic of having a high rate of STD and non-default.
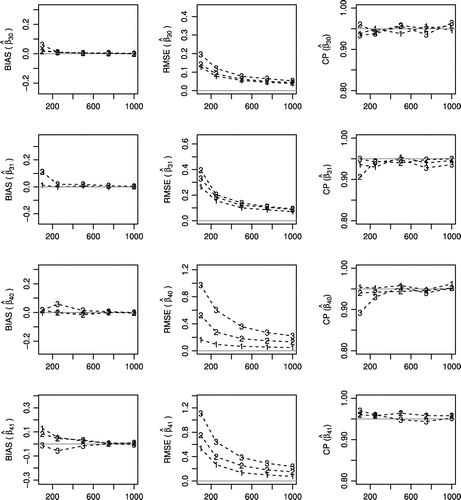
Figure 4. MLEA, maximum likelihood estimation on average of the parameters ,
,
,
,
,
,
,
of zero-inflated Promotion Cure rate regression model for simulated data under the three scenarios of parameters, obtained from Monte Carlo simulations with 1,000 replications and increasing sample size (n). 1 indicates the scenario 1 with characteristic of having a low rate of STD and non-default. 2 indicates scenario 2 with characteristic of having a moderate rate of STD and non-default. 3 indicates the scenario 3 with characteristic of having a high rate of STD and non-default.
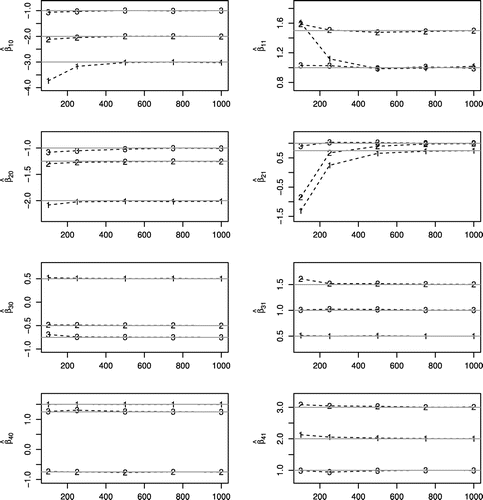
Table 1. Frequency and percentage of the bank loan lifetime data
Table 2. Quantity of the available covariates
Figure 5. Brazilian bank loan portfolio data.
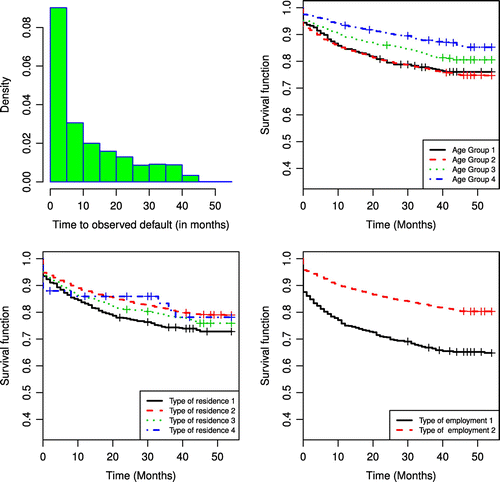
Table 3. The zero-inflated promotion cure regression model for time to default on a Brazilian bank loan portfolio
Figure 6. Brazilian bank loan portfolio. Kaplan–Meier survival curves stratified through the covariate selection given by the final promotion cure rate regression model presented in the Table .
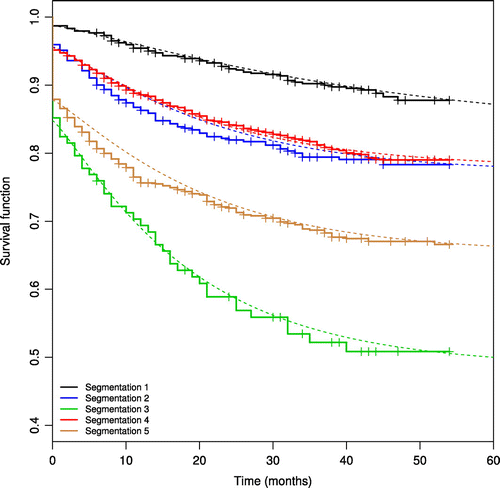