Figures & data
Figure 1. Mapping characteristics based on four kinds of kernel functions (a) RBF kernel function, (b) polynomial kernel function, (c) linear kernel function, and (d) sigmoid kernel function.
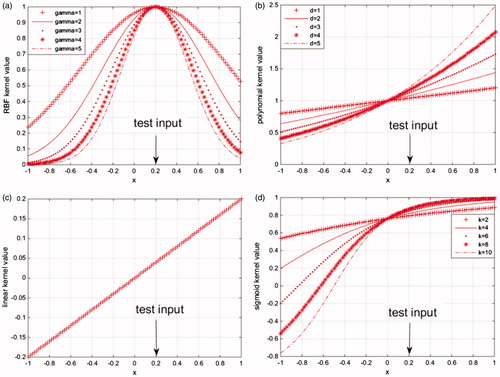
Figure 2. Mapping characteristics based on three kinds of mixed kernel functions (a) RBF kernel mixes with linear function, (b) RBF kernel mixes with polynomial kernel function, and (c) RBF kernel mixes with sigmoid kernel function.
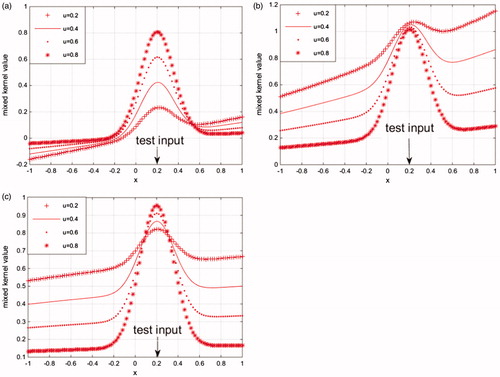
Table 1. Main characteristics of datasets.
Table 2. The optimization parameters c, g, u of PSO-RBF kernel, PSO-mixed kernel and improved PSO-mixed kernel SVM and classification accuracy rate of the test set.