Figures & data
Figure 1. (A) B-mode ultrasound images while recording echo signals. (B) Acoustic nonlinearity map was composed of 4 channels derived from 4 imaging functions (Q1, Q2, Q3 and Q4) with a total of 740 * 110 * 4 pixels. The region of interest (ROI) with size of 2 cm * 2 cm was positioned 1 cm below the liver capsule in each acoustic nonlinearity map.
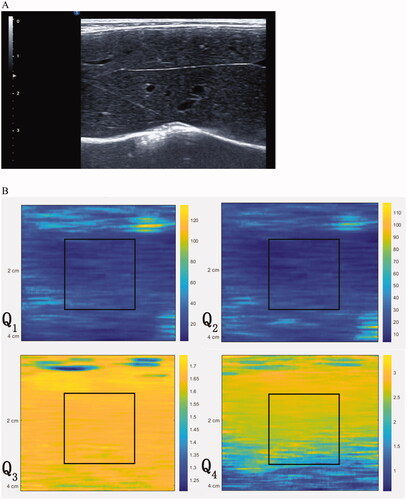
Figure 2. Illustration of construction of deep learning networks. CNN consisted of feature extraction network and classification network. Four convolutional layers and 2 max pooling layers were contained in feature extraction network. In classification network, softmax function was employed for the final fully connected layer. The model of CNN combined with classifiers consisted of feature extraction network from CNN and classifiers network. Classifiers such as SVM, random forest or logistic regression can be found in classifiers network.
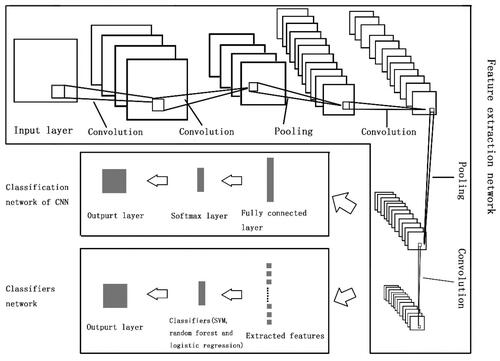
Table 1. Hyperparameters considered in CNN.
Figure 3. Nested 10-fold cross-validation procedure for hyperparameter selection and model evaluation.
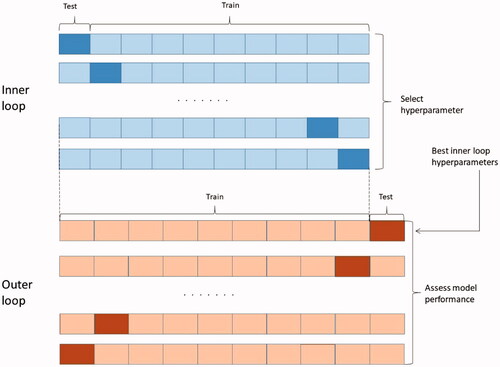
Figure 5. Structure of CNN in the study. The padding mode in each convolutional layer and pooling layer was ‘same’.
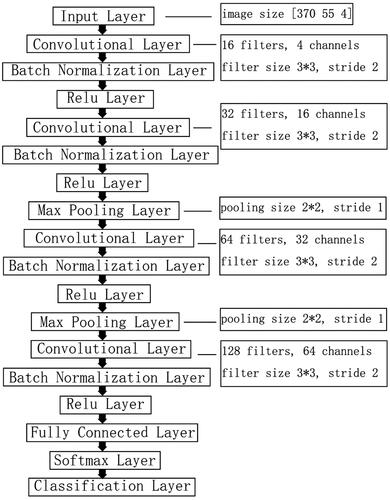
Figure 6. Comparison of receiver operating characteristic (ROC) curves among CNN, CNN + SVM, CNN + random forest and CNN + logistic regression. (A) ROC curve for classifying significant fibrosis (≥F2); (B) ROC curve for classifying advanced fibrosis (≥F3); (C) ROC curve for classifying cirrhosis (F4).
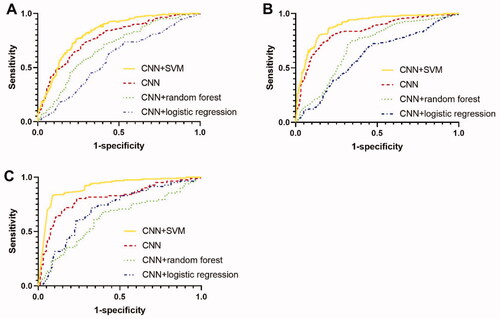
Table 2. Comparisons using different models of deep learning to classify liver fibrosis stages.
Table 3. Confusion matrix.