Figures & data
Figure 1. IG construction strategy from majority class samples, minority class samples are preserved (Chen et al., Citation2008).

Figure 2. IG description: the concept of ‘sub-attributes’ used for numeric attribute (Chen et al., Citation2008).
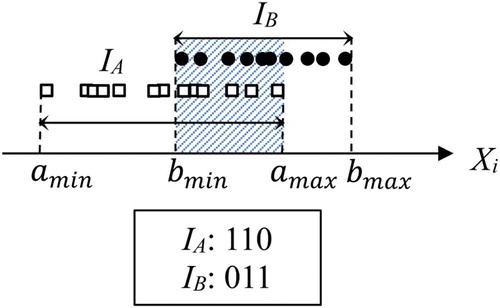
Figure 4. IG description: the concept of ‘sub-attributes’ (a) using for discretization of numeric attributes (number of discretized values is 10) and (b) for discrete attributes Xj (8 distinct values).
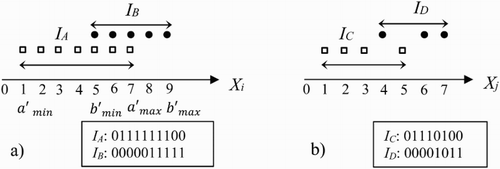