Figures & data
Table 1 MCI Studies Variable Assignment
Table 2 Single Factor Analysis of the Influence of Lifestyle on MCI
Table 3 Binary Logistic Regression Analysis of the Influence of Lifestyle on MCI
Figure 1 Decision tree model of the influence of lifestyles on MCI. This tree is generated by the software through 10 cross-validations. Each box is numbered sequentially. The blue icon represents MCI, and the red icon represents NC. Each box has its own percentage and specific number of examples. Below each box is the influence factor of the next classification; at the top of each box is the corresponding category of influence factor.
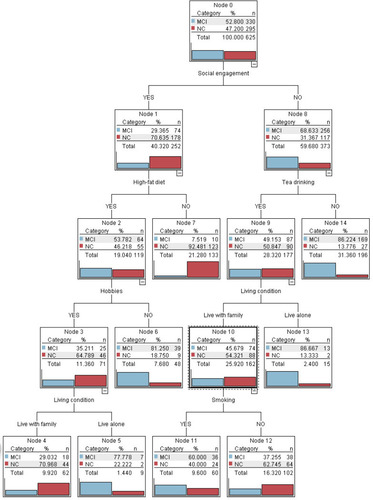