Figures & data
Table 1 Different types of confounders and potential solutions on how to control for these in observational studies based on health care databases
Table 2 Examples of instrumental variables recently used in published studies
Figure 1 First CD4 count and mortality hazard rate in an HIV-positive population.
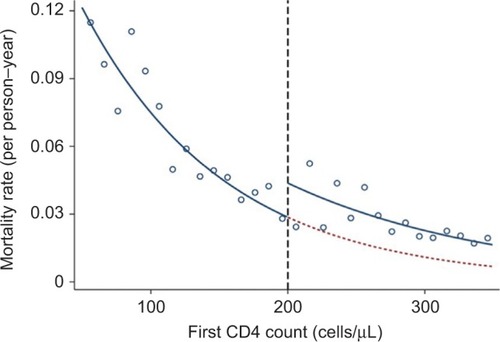
Figure 2 Causal diagram showing an ideal negative control exposure B for use in evaluating studies of the causal relationship between exposure A and outcome Y.
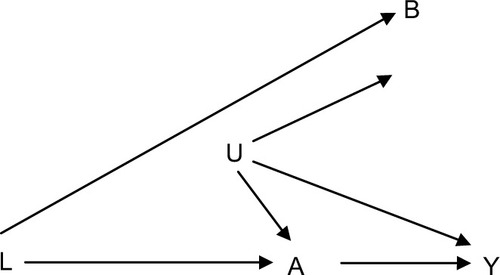