Figures & data
Figure 1 Deriving the outcome variables. This exemplary admission is composed of three successive in-patient visits (ie the patient has been transferred twice represented by the arrows). The admission is eligible because it spans more than 24 hours and an eGFR ≤30 was measured before index. Here, apixaban was given while the patient’s eGFR was ≤30, but dose reduction rendered these administrations appropriate.
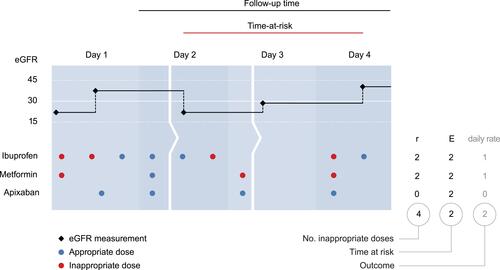
Table 1 Univariate Summary Statistics of Select Features. Values are Median (Inter-Quartile Range) and Count (Proportion) as Appropriate. Distinct Patients and Distinct Women Show Counts of Actual Patients (as a Patient Can Contribute More Than One Unit)
Table 2 Performance Metrics of Final Models and Results of Optuna Hyperparameter Optimization
Figure 2 Bivariate relationships between values of select features (x axis) and their corresponding shap values (y axis). The continuous features are summarized by locally estimated scatterplot smoothing (LOESS), binary features by vertical density bands.
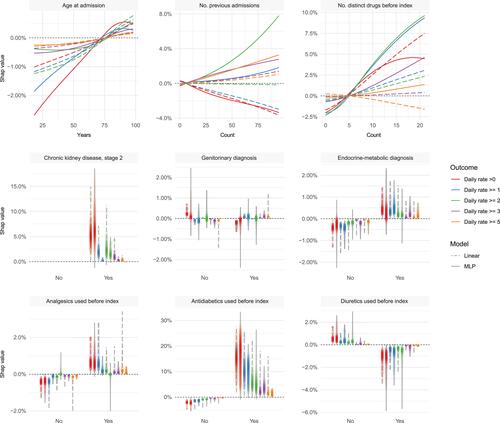