Figures & data
Table 1 Characteristics of Patients with LA-NPC in the Training and Validation Cohorts
Table 2 Coefficient Profiles, Category and Filters of the 20 Radiomics Features Selected by LASSO-Logistic
Table 3 Performance of Radiomics Nomogram, Clinical Nomogram and TNM Staging System
Figure 1 Framework of the study (A) Regions of interest (ROI). (B) Three types of features. (C) Feature selection by T-test and Lasso-logistic regression. (D) Establishment and validation of radiomics signature. (E) Establishment and validation of Nomogram.
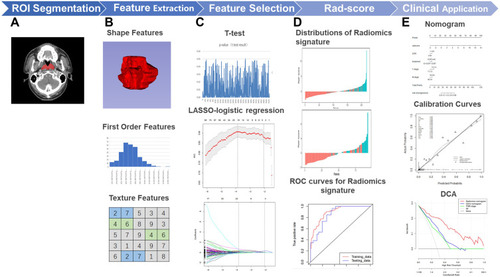
Figure 2 LASSO-logistic regression analysis for selection of radiomics features and the distribution of radiomics signature (A) The tuning parameters (λ) in Lasso-Logistic regression were selected by 10-fold cross-validation. When log(λ) is equal to −3.87, the AUC reaches its maximum value. (B) LASSO coefficient profiles of 527 radiomics features. 20 features with non-zero coefficients are selected at the optimal λ. Rad-score distribution of (C) patients in the training cohort and (D) patients in the validation cohort. Red bars show scores for patients were progression-free; green bars show scores for patients who experienced tumor progression or death by any cause.
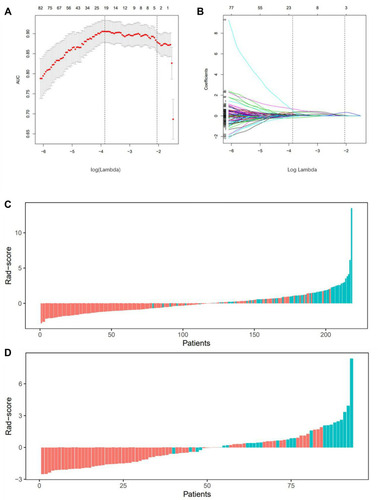
Figure 3 Establishment of Nomograms. (A) Radiomics nomogram, (B) Clinical nomogram and (C)TNM staging model.
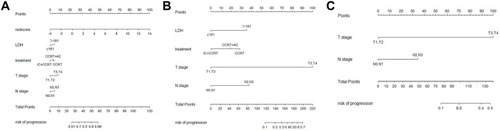
Figure 4 Validation of Nomograms. The ROC curves of three models for (A) The training cohort and (B) the validation cohort. The Calibration curves of radiomics nomogram for (C) the training cohort and (D) the validation cohort. The Calibration curves are close to the standard curves, suggesting that the model has high accuracy in both cohorts. Decision curve analysis (DCA) for the radiomics nomogram and clinical nomogram of (E) the training cohort and (F) the validation cohort. The DCA indicates that radiomics nomogram provide more net benefit than the clinical nomogram and TNM staging model with threshold probability in both cohorts.
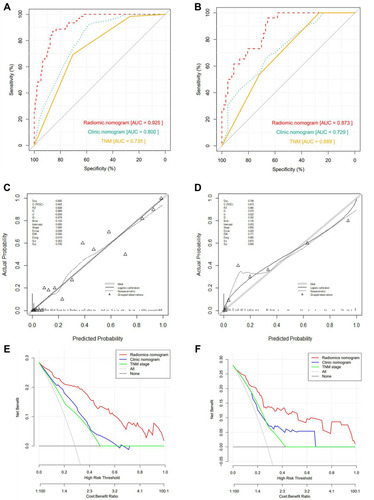