Figures & data
Table 1 Univariate Logistic Regression Analyses of COPD Influencing Factors
Figure 1 Screening predictors using LASSO binary logistic regression model.
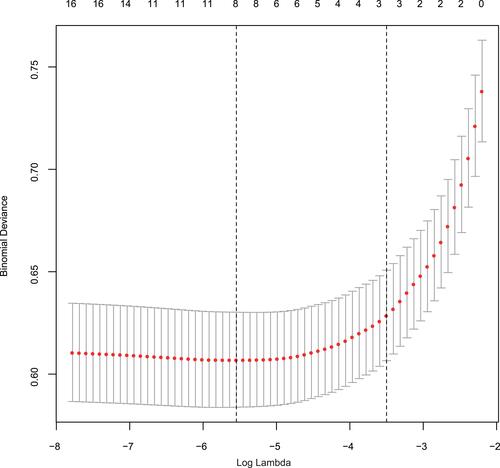
Figure 2 A nomogram to predict the development of COPD.
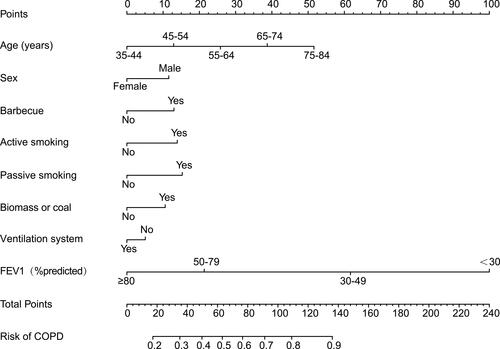
Figure 3 Calibration curve to predict the development of COPD.
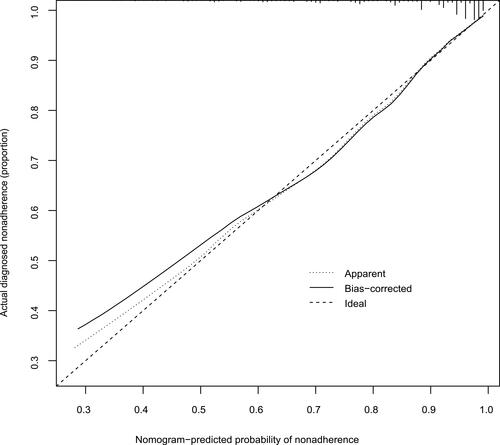