Figures & data
Figure 1 Data screening flow chart.
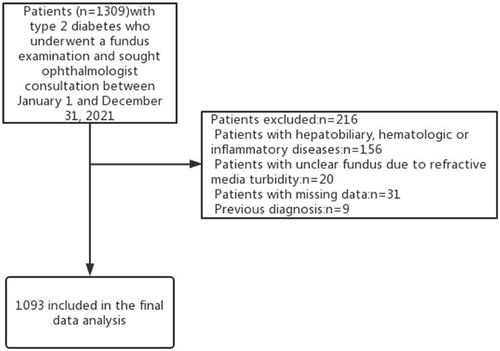
Table 1 DR Dataset
Figure 4 An association between classification accuracy (ACC) and number of distinct hidden neurons in the ELM (database of our hospital).
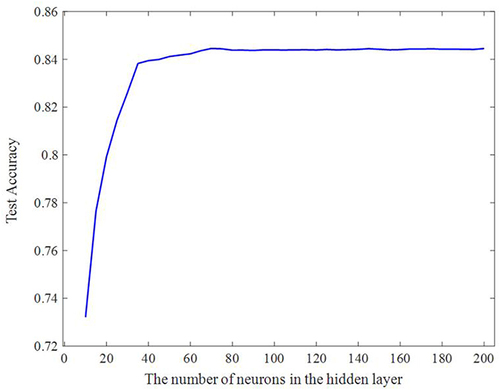
Table 2 The Extreme Learning Model (ELM) Model Produced Detailed Findings
Table 3 Extreme Learning Machine, Support Vector Machine, Artificial Neural Network, Random Forest, and k-Nearest Neighbor (ELM, SVM, ANN, RF, and KNN, Respectively) Classification Performances Were Compared