Figures & data
Table 1 The Criteria of the International Diabetes Federation (IDF) for the Definition of Metabolic Syndrome (MetS)
Table 2 The Missing Percentages of Each Variable
Table 3 Baseline Characteristics of Sub-Groups from Patient Cohorts
Table 4 Performance Metrics of Machine-Learning Models Using Longitudinal Data
Figure 2 ROC curves of the three models for all the datasets.
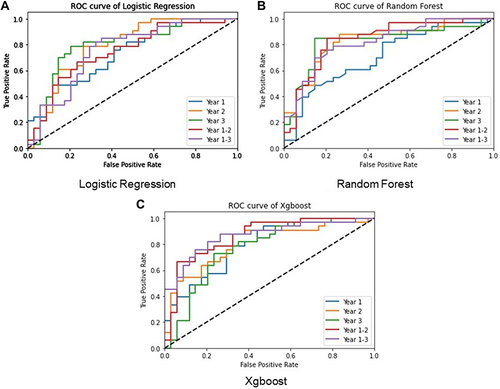
Figure 3 Feature importance of each dataset using LASSO.
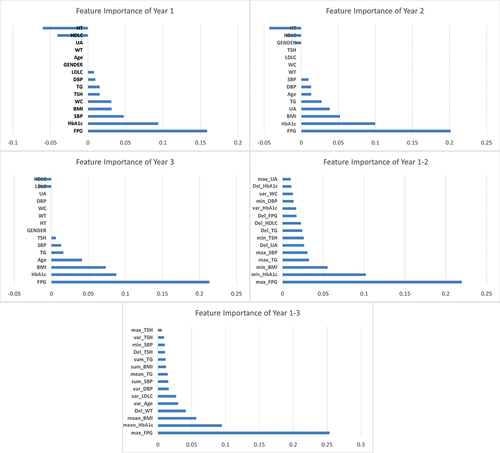