Figures & data
Table 1 Baseline Characteristics of the Modeling Group and Validation Groupa
Table 2 Univariate Analysis Between Survivors and No Survivors in Modeling Groupa
Table 3 Stepwise Regression Analysis of Independent Risk Factors for Death in Patients with Septic Shock
Figure 1 The nomogram of established model for predicting mortality risk in septic shock patients during hospitalization. The enrolled variables were collected for the first time after admission. A patient was displayed as an example, with detailed enrolled variables labelled by red dots. The variables labelled of asterisk indicated significance in the model, *P < 0.05, **P < 0.01, ***P < 0.001.
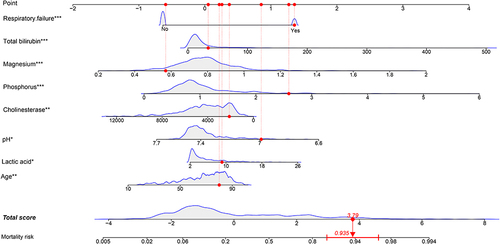