Figures & data
Table 1 Demographic and Clinical Factors of AKI in Patients with Hepatobiliary Malignancies
Figure 1 (A) Importance matrix plot of AKI predictors in XGBoost model among liver cancer patients. (B) Importance matrix plot of AKI predictors in XGBoost model among gallbladder cancer patients.
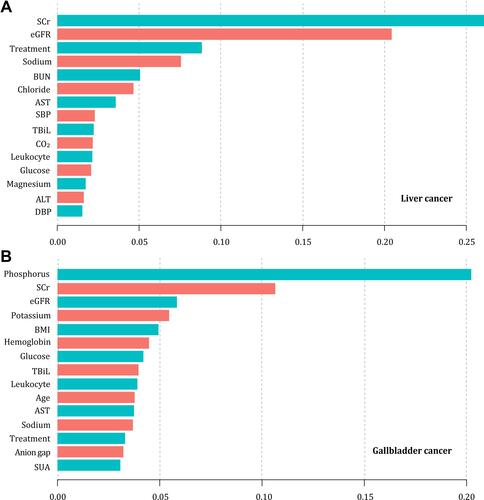
Table 2 Predictive Variables of AKI Selected by LASSO-Logistic Regression
Table 3 External Validation of AKI Prediction Models Based on XGBoost and LASSO-Logistic Algorithms
Figure 2 (A) Receiver operating characteristic curves of AKI prediction models among liver cancer patients; (B) receiver operating characteristic curves of AKI prediction models among gallbladder cancer patients; (C) left chart of AKI prediction models among liver cancer patients; (D) left chart of AKI prediction models among gallbladder cancer patients; (E) learning curve of AKI prediction models among liver cancer patients; (F) learning curve of AKI prediction models among gallbladder cancer patients.
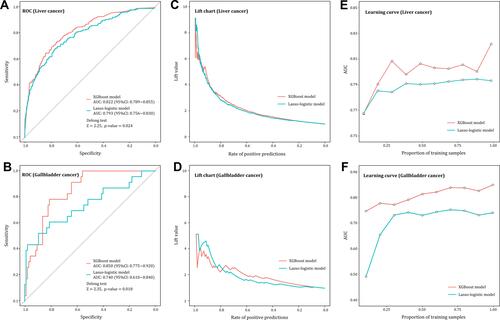