Figures & data
Table 1 Demographics and Clinical Characteristics of the DGF and Non-DGF Groups
Table 2 Morbidity of DGF Prediction Factors After Renal Transplantation from DD Donors
Table 3 Evaluation Results for the Proposed Model Among 10 Randomly Selected Independent Subgroups
Figure 2 Demographics and clinical features selection using the LASSO binary regression model.
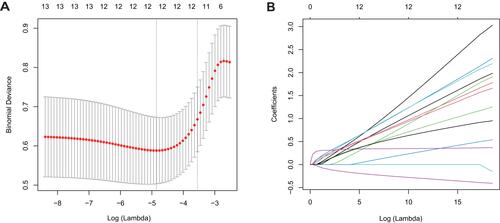
Figure 3 Developed nomogram of DGF.
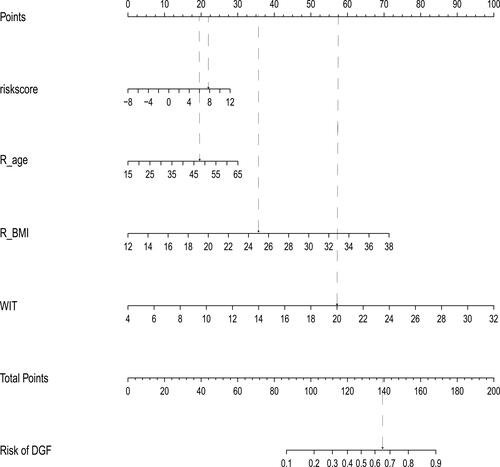
Figure 4 Assessment of the clinical effectiveness of the proposed model.
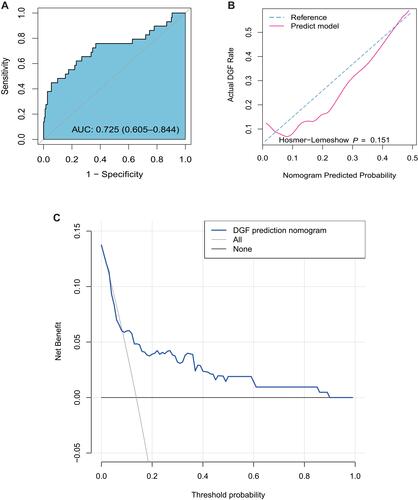