Figures & data
Table 1 Clinical Characteristics for Different Cohorts
Figure 1 Identification of differentially expressed pyroptosis-related genes and association with molecular subtypes. (A) Experimental flow chart. (B) PCA plot of gliomas and normal cerebral cortex from UCSC database. (C) Barplot of expression of differentially expressed pyroptosis-related genes between gliomas and normal samples (“***” represented p-value < 0.001). (D) Heatmap of consensus clustering matrix of 589 glioma patients based on the 15 differentially expressed pyroptosis-related genes in the TCGA cohort (k = 2). (E) The heatmap shows the level of expression of 15 differentially expressed genes between two subgroups and the association between clinical traits. (F) PCA plot of consistent clustering results. (G) Kaplan–Meier survival curves of c1 and c2 subgroups.
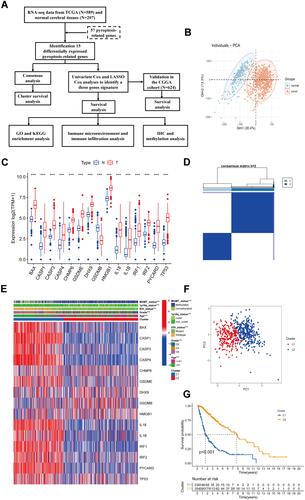
Figure 2 Identification of risk signature genes. (A) Univariate Cox regression analysis for all differentially expressed pyroptosis-related genes and obtained 13 prognostic genes with the criterion of p < 0.05. (B) Distribution of LASSO coefficients of 13 candidate genes. (C) 10-fold cross-validation for tuning the parameter selection in the LASSO regression model. (D) ROC curves of risk signature for predicting IDH status, 1p19q status, MGMT status, and clustering.
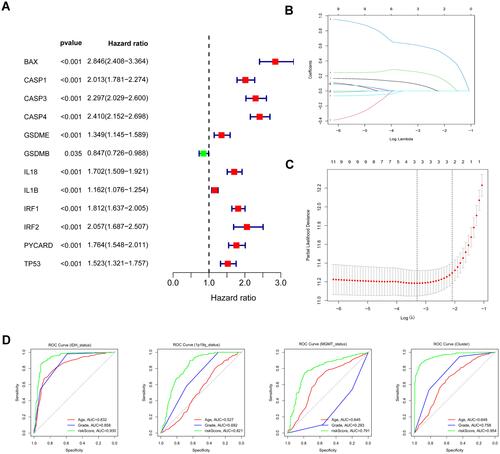
Figure 3 Construction and survival analysis of risk signature in the TCGA cohort. (A) Distribution of survival status of 589 patients from the TCGA cohort. (B) Heatmap of the expression of three risk genes in the TCGA cohort. (C) PCA plot showed the distribution of glioma patients based on the risk signatures. (D) Kaplan–Meier survival curves for comparison of survival between low and high-risk subgroups in the TCGA cohort. (E) The time-dependent ROC curve of 1-, 3-, and 5-year and their AUCs. (F) ROC curve of risk score and other indicators at 5 years in the TCGA cohort.
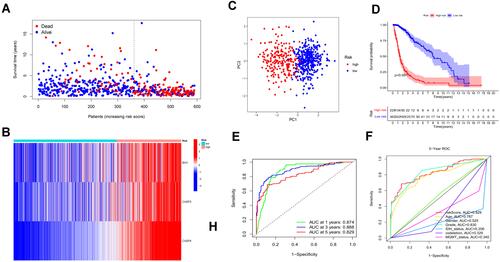
Figure 4 Validation of the prognostic model in the CGGA cohort. (A and B) Survival status and the expression of three risk genes of patients between low and high-risk groups. (C) PCA showed the distribution of primary gliomas in the CGGA cohort. (D) KM survival curve of overall survival in the testing set. (E) Time-dependent ROC curve and AUC values for estimating the efficiency of the risk model at 1-, 3-, 5-year. (F) ROC curve of risk score and other indicators at 5 years in the testing set.
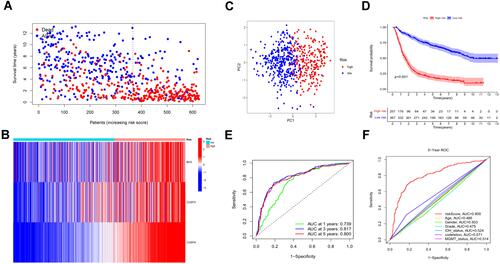
Figure 5 Univariate and multivariate independent prognostic analysis of risk score signature. (A and C) Univariable Cox regression analyses of the risk signature and clinical traits with p < 0.05 were considered statistically significant in the TCGA and CGGA cohorts. (B and D) Forest plot of multivariate Cox regression analysis of risk score and clinical characteristics in the training and testing sets.
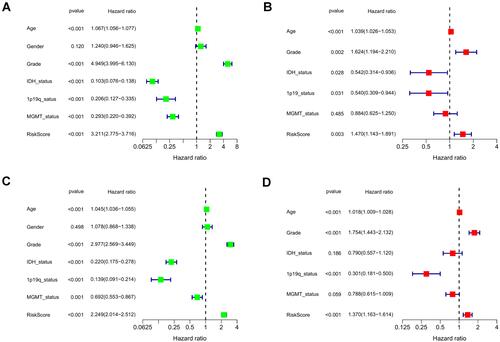
Figure 6 Association of risk score with various clinical traits. (A and B) Stratified analysis of risk score with various clinicopathological parameters in glioma patients in the TCGA and CGGA cohorts such as age, grade, IDH status, 1p19q codeletion status, and MGMT methylation status.
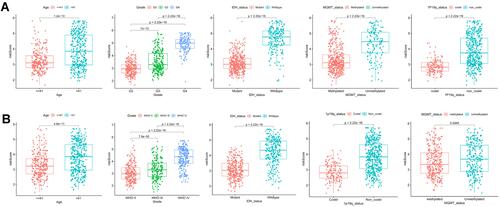
Figure 7 Construction of nomogram for predicting overall survival. (A) Nomogram constructed with the risk score and clinical characteristics to predict the probability of 1-, 3-, 5-year survival of patients in the train set. (B and C) Calibration curves of the nomograms for evaluation of the consistency between actual and predicted os times in 1, 3, 5 years. (D and E) ROC curves of nomogram at 1, 3, and 5 years in the training and testing set respectively.
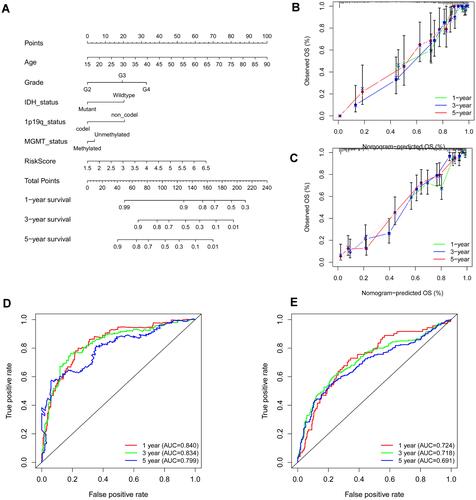
Figure 8 Functional analysis based on the DEGs between low and high-risk subgroups in the TCGA cohort. (A) Analysis of biological progress enrichment. (B) Analysis of KEGG enrichment.
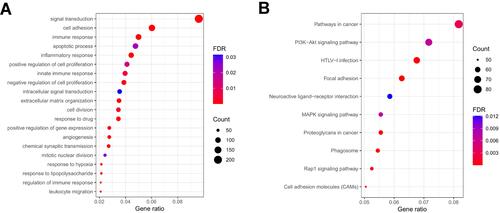
Figure 9 Tumor microenvironment and immune analysis of risk model. (A) Correlation scatters plot of risk score and tumor microenvironment score in the training set. (B) Boxplot showed the differences in absolute infiltration score of 22 immune cells calculated by CIBERSORT between the low and high-risk glioma populations. (C) Correlation of BAX, CASP3, CASP4, and 22 immune cells. (D) Difference of 28 immune cells between two risk subgroups calculated by ssGSEA. (E) Correlation of MDSC with the risk score. (F) Difference of tumor-associated chemokine, immunosuppressive cytokine, and immune checkpoint genes in high and low-risk groups. (“***” represented the p-value < 0.001).
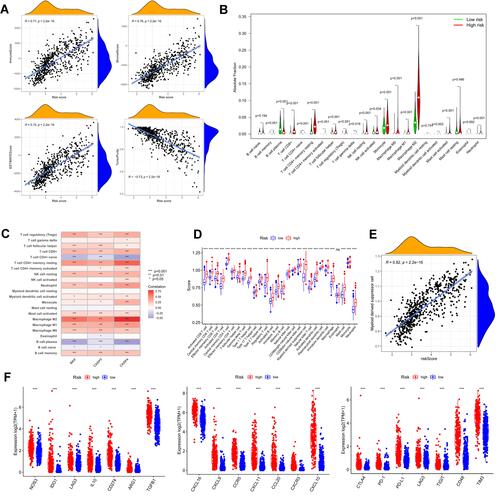
Figure 10 The expression of BAX and CASP3 and CASP4 in different grades of gliomas. (A) The expression of the three pyroptosis genes in glioma patients with different grades in the TCGA cohort. (B)–(D) The protein expression level of BAX and CASP3 and CASP4 in the glioma tissues with grades 2, 3, 4 by immunohistochemistry assay (E), *** represented p < 0.001, ** represented p <0.01 and * represented p <0.05).
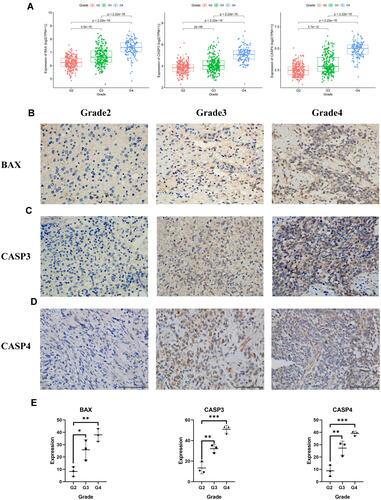