Figures & data
Figure 1 Fully automated hybrid model for HE prediction. In Model 2, we use feature-level fusion approaches for fusion of features with the AIM of collecting complementary information from radiomics, clinical data.
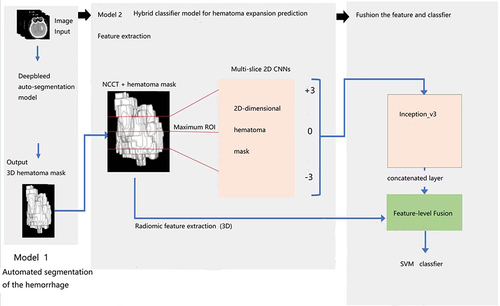
Table 1 The Characteristics of All Inclusion Participants
Figure 3 Representative image of hematoma by automatically labeled. The effect of the automatic hematoma labeling tool on a certain patient. The red color in the above picture shows the range of the labeled hematoma, and the picture below shows the original axial image of the brain.
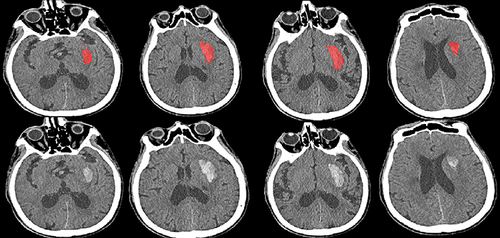
Table 2 Results of Hybrid Module Receiver Operating Characteristic, Precision-Recall