Figures & data
Table 1 Univariate and Multivariate Analyses of Variables Related to Postoperative Myocardial Injury
Figure 2 The variable influence factor ranking plots of the four models. (A) Variable importance ranking diagram of the XGBoost model. (B) Variable importance ranking diagram of the RF model. (C) Variable importance ranking diagram of the MLP model. (D) Variable importance ranking diagram of the KNN model.
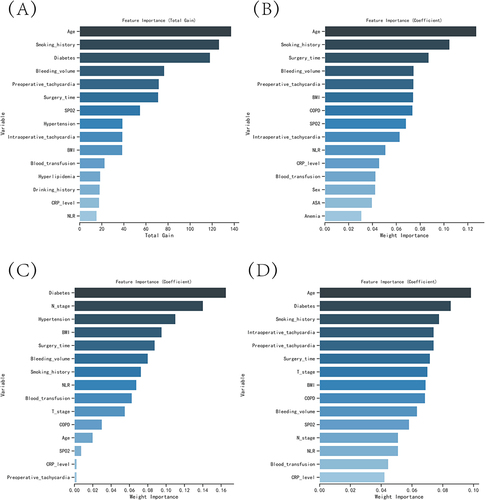
Table 2 Evaluation of the Four Models
Figure 3 Evaluation of the four models for predicting myocardial injury. (A) ROC curves for the training set of the four models. (B) ROC curves for the validation set of the four models. (C) Calibration plots of the four models. In a calibration plot, the 45° dotted line represents perfect calibration, where the observed and predicted values are perfectly matched. The distance between the observed and predicted values is shown by the curves, and a closer distance indicates greater accuracy of the model. (D) DCA curves of the four models. In a decision curve analysis, the x-axis represents the threshold probability of the outcome, while the y-axis represents the net benefit of making a decision based on the model’s prediction. The “All” curve represents the net benefit of treating all patients, regardless of the model’s prediction, while the “None” curve represents the net benefit of not treating any patients.
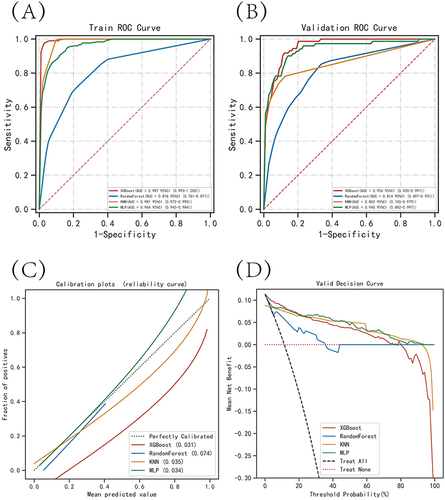
Figure 4 Internal validation of the XGBoost model. (A) ROC curve of the XGBoost model for the training set. (B) ROC curve of the XGBoost model for the validation set. (C) ROC curve of the XGBoost model for the test set. (D) External validation of the XGBoost model.
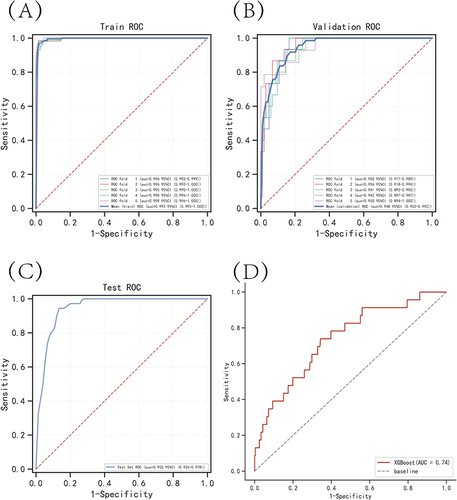
Figure 5 SHAP summary plot. Risk factors are arranged along the y-axis based on their importance, which is given by the mean of their absolute Shapley values. The higher the risk factor is positioned in the plot, the more important it is for the model.
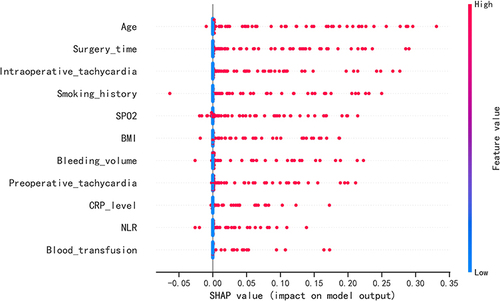
Figure 6 SHAP force plot. SHAP analysis is a method used to explain the output of a machine learning model by attributing the contribution of each feature to the final prediction. The SHAP values represent the contribution of each feature to the difference between the actual prediction and the baseline prediction. In the horizontal line plot, each feature is represented by a bar with a corresponding SHAP value. The position of the bar along the horizontal line indicates the magnitude and direction of the impact of the feature on the prediction. The features are sorted by their absolute SHAP value, with the most important features on the left and the least important on the right. The color of the bar represents the direction of the feature’s impact. Blue bars indicate features that have a negative effect on the disease prediction, meaning that an increase in the feature value decreases the likelihood of disease. Red bars indicate features that have a positive effect on disease prediction, meaning that an increase in the feature value increases the likelihood of disease. (A) Predictive Analysis of Patient I. (B) Predictive Analysis of Patient II.
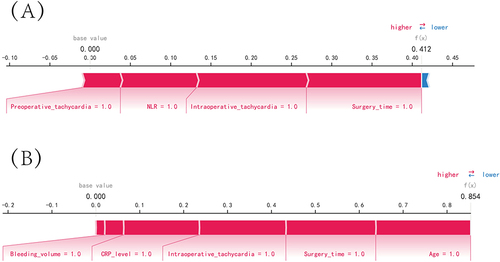
Data Sharing Statement
The original data presented in the study are included in Supplementary Material, and further inquiries can be directed to the corresponding author ([email protected]).