Figures & data
Figure 1 This figure illustrates the systematic processing of the data records through various AI models. The journey begins with raw data inputs like patient records or medical imagery directed towards specialized AI models such as Artificial Neural Networks (ANN), Machine Learning (ML), Natural Language Processing (NLP), and Computer Vision (CV). The extracted information is then structured and organized, allowing for a coherent interpretation.
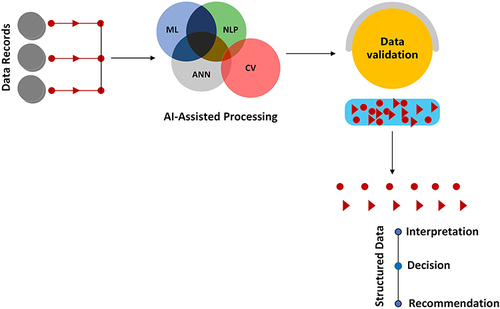
Figure 2 AI-enhanced workflow for feto-maternal health: The process involves rigorous data preprocessing, feature extraction, and AI model training for precise medical diagnoses. Iterative feedback loops, including real-time fetal monitoring and risk assessment with tailored treatment recommendations. In cases of inconclusive results, a dynamic feedback mechanism directs the data back to the AI model training or medical diagnosis steps, or even to reconsider the patient data, ensuring continuous refinement until conclusive outcomes are achieved.
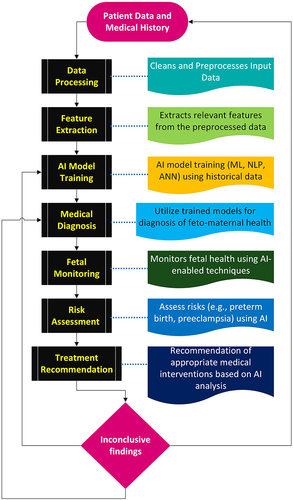
Figure 3 The figure depicts the number of studies identified on PubMed and IEEE Xplore using the keyword “Artificial Intelligence in Pregnancy”: The presentation of data aims to illustrate an overall trend in these studies. It’s crucial to note that the publication numbers serve as an indicative list and may not fully represent the entire volume of publications. This count specifically includes studies conducted on human subjects (women) and has been identified with a single keyword. The count on IEEE Xplore includes both conference papers and journal articles, while the count on PubMed is limited to journal articles alone.
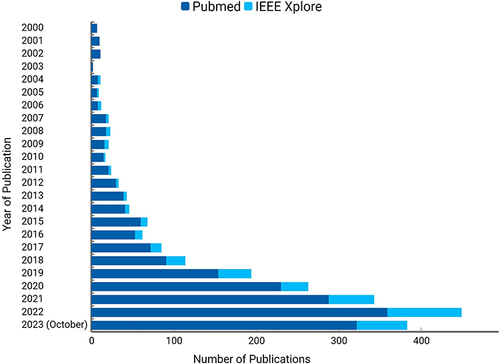